video
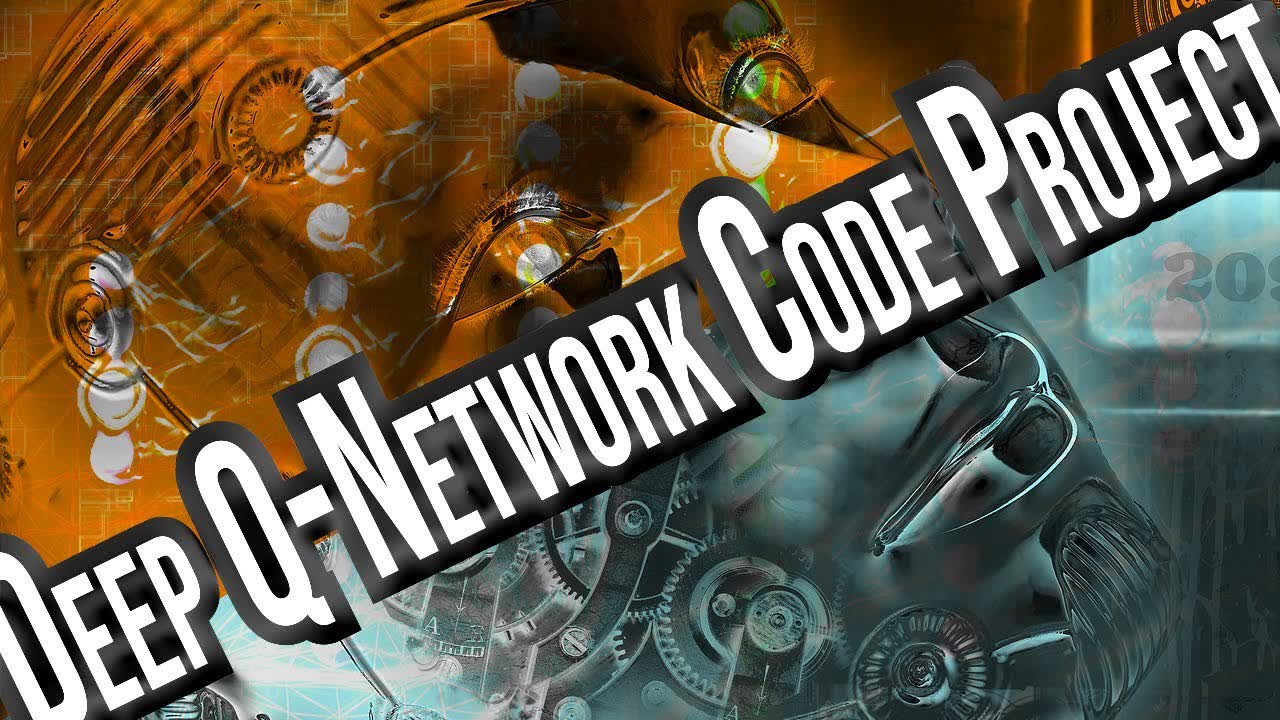
Deep Learning Course - Level: Advanced
Welcome back to this series on reinforcement learning! It's finally time to apply everything we've learned about deep Q-learning to implement our own deep Q-network in code! In this episode, we'll get introduced to our reinforcement learning task at hand and go over the prerequisites needed to set up our environments to be ready to code. Let's get to it!
Committed by on