video
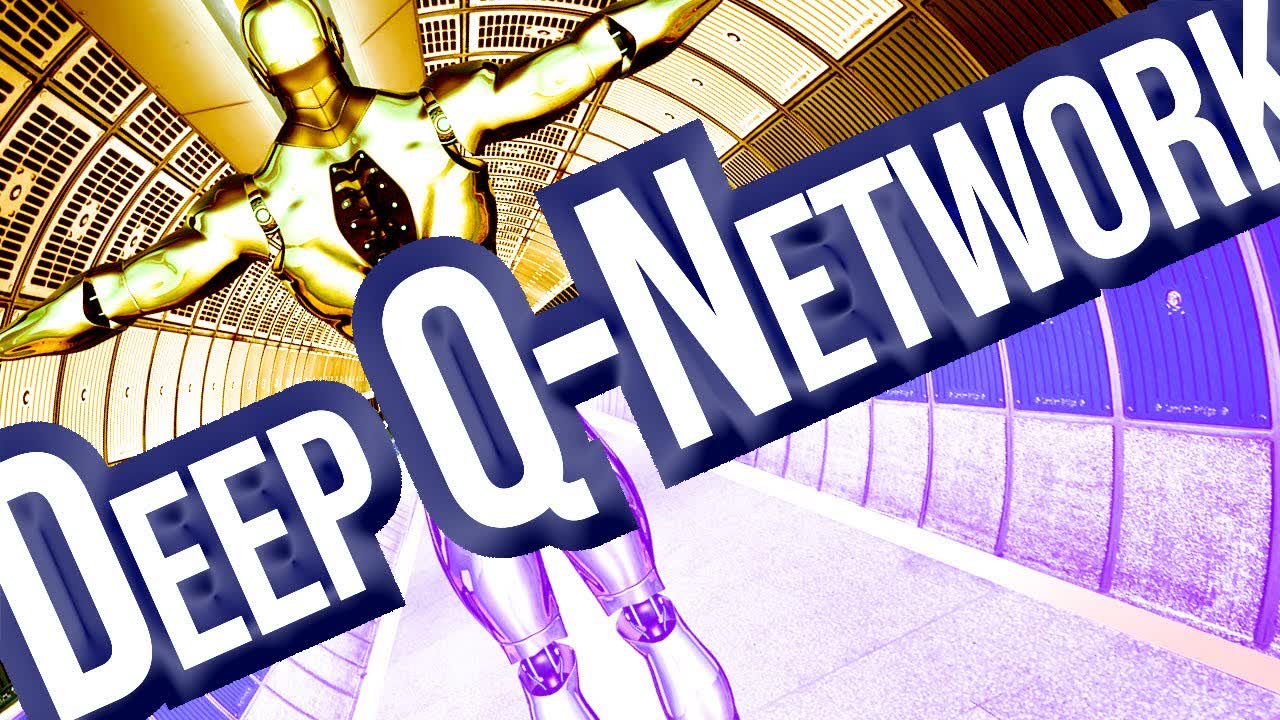
Deep Learning Course - Level: Advanced
Welcome back to this series on reinforcement learning! In this episode, we'll get started with building our deep Q-network to be able to perform in the cart and pole environment. Let's get to it!
After following the environment prep we covered last time, we're now ready to start writing our code. We'll be making use of everything we've learned about deep Q-networks so far, including the topics of experience replay, fixed Q-targets, and epsilon greedy strategies, to develop our code.
Committed by on