video
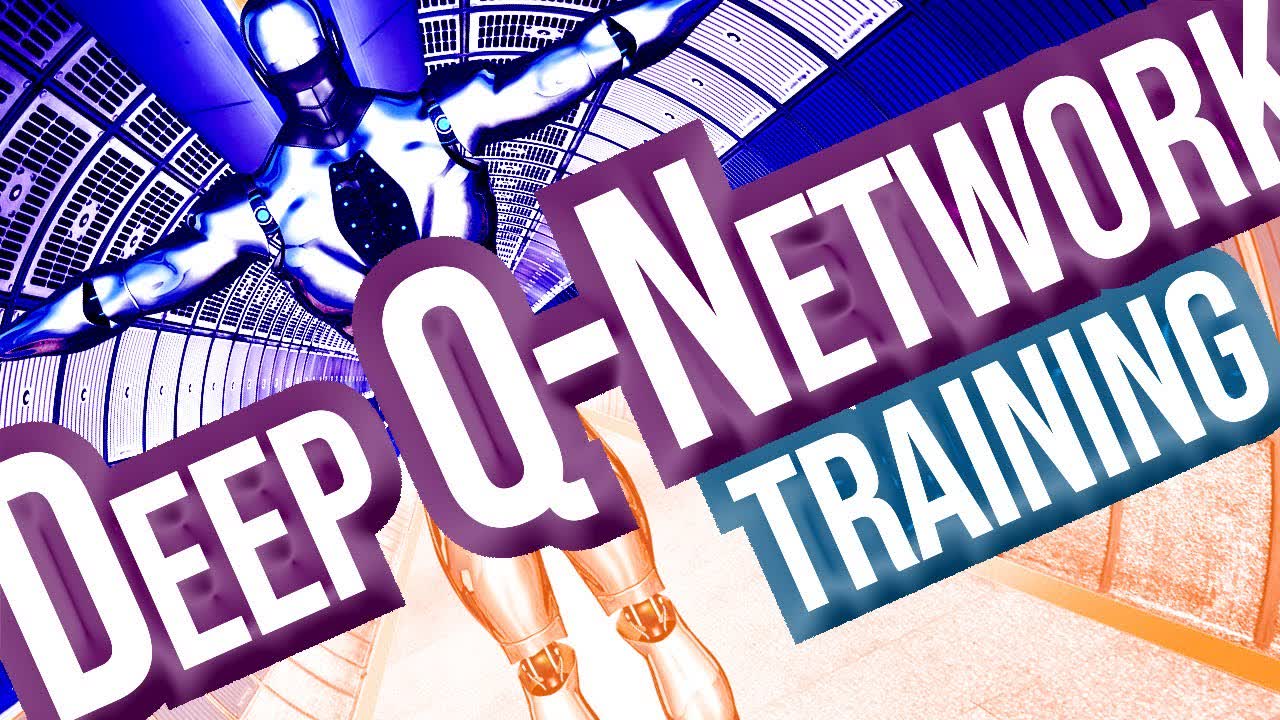
Deep Learning Course - Level: Advanced
Welcome back to this series on reinforcement learning! In this episode we'll be bringing together all the classes and functions we've developed so far, and incorporating them into our main program to train our deep Q-network for the cart and pole environment.
Then, we'll see the training process live as we watch our agent's ability to balance the pole on the cart increase as it learns.
Committed by on