video
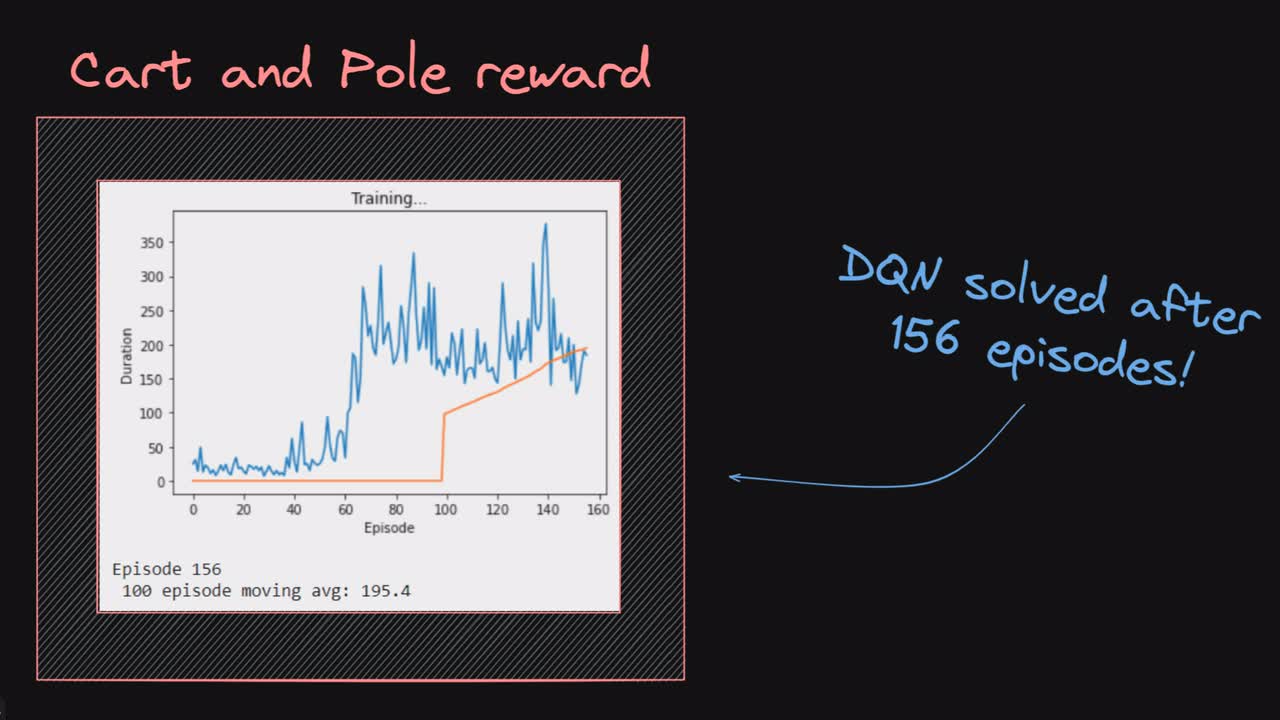
Deep Learning Course - Level: Advanced
Welcome back to this course on reinforcement learning! In this episode, we'll discuss how we can tune our current code project in order for our deep Q-network to solve the Cart and Pole environment.
We last left off having completed our program by training our deep Q-network on the Cart and Pole environment. There, we saw that the network's average reward consistently grew over the 1000
episode training duration, with its final score reaching 100
-episode average reward of 90
.
Committed by on