video
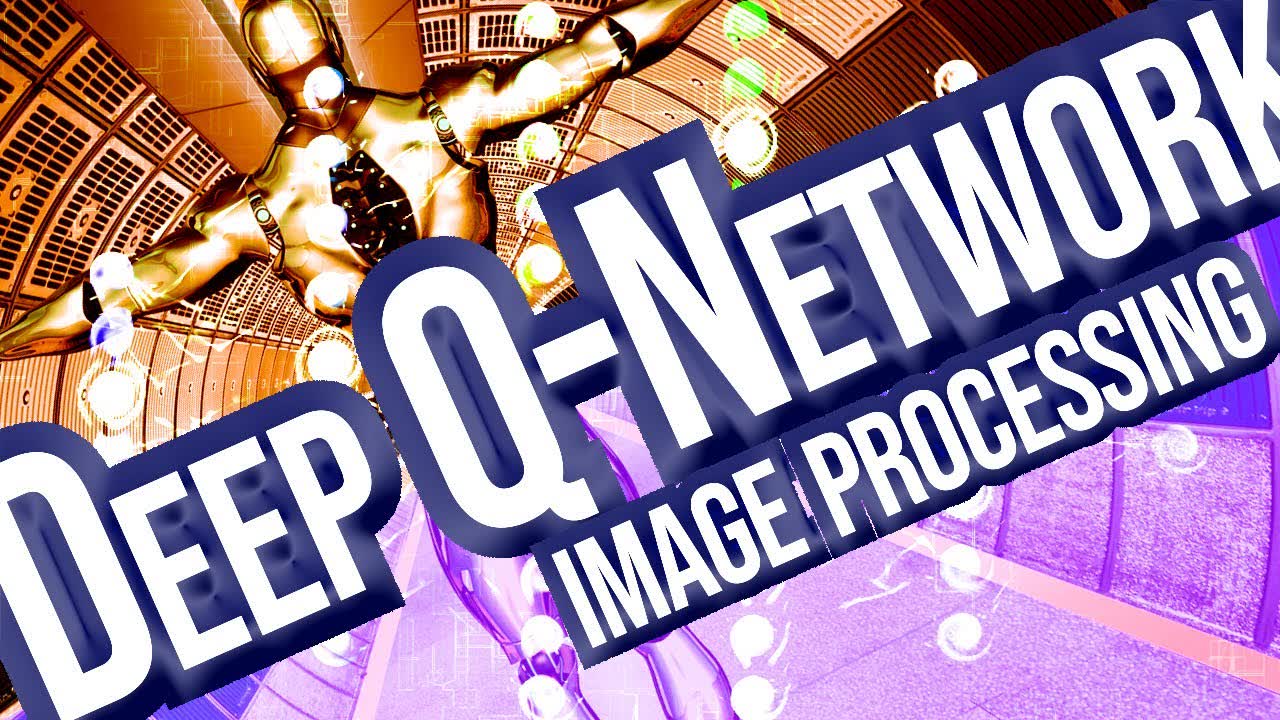
Deep Learning Course - Level: Advanced
Welcome back to this series on reinforcement learning! In this episode, we'll be continuing to develop the code project we've been working on to build a deep Q-network to master the cart and pole problem. We'll see how to manage the environment and process images that will be passed to our deep Q-network as input.
Committed by on