Save and Load a Model with TensorFlow's Keras API
text
Save and load a model with TensorFlow's Keras API
In this episode, we'll demonstrate how to save and load a tf.keras.Sequential
neural network.
There are a few different ways to save a Keras model. The multiple mechanisms each save the model differently, so we'll check them all out.
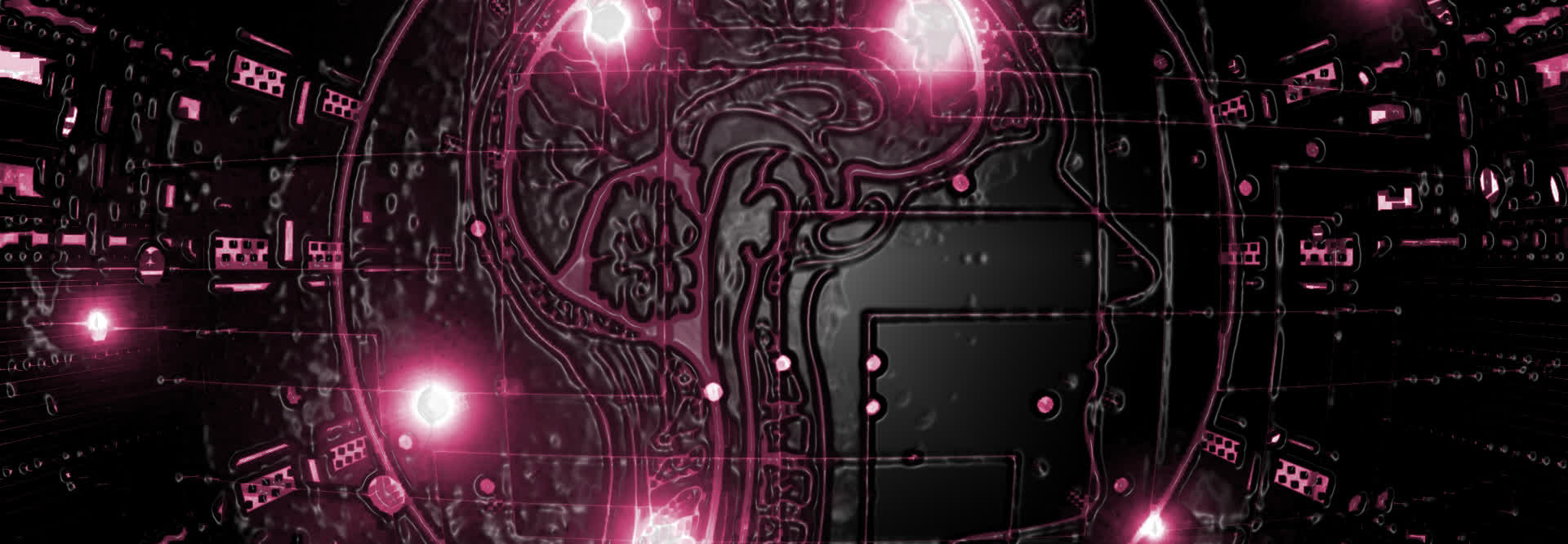
In a previous episode, we created and trained this model.
model = Sequential([
Dense(units=16, input_shape=(1,), activation='relu'),
Dense(units=32, activation='relu'),
Dense(units=2, activation='softmax')
])
We'll work with this model to demonstrate the different saving and loading techniques.
Saving and loading the model in its entirety
If we want to save a model at its current state after it was trained so that we could make use of it later, we can call the save()
function on the model. To save()
, we pass in the
file path and name of the file we want to save the model to with an h5
extension.
model.save('models/medical_trial_model.h5')
Note, this function also allows for saving the model as a Tensorflow SavedModel
as well if you'd prefer.
This method of saving will save everything about the model β the architecture, the weights, the optimizer, the state of the optimizer, the learning rate, the loss, etc.
Now that we have this model saved, we can load the model at a later time.
To do so, we first import the load_model()
function. Then, we can call the function to load the model by pointing to the saved model on disk.
from tensorflow.keras.models import load_model
new_model = load_model('models/medical_trial_model.h5')
We can verify that the loaded model has the same architecture and weights as the saved model by calling summary()
and get_weights()
on the model.
new_model.summary()
Model: "sequential_1"
_________________________________________________________________
Layer (type) Output Shape Param #
=================================================================
dense_1 (Dense) (None, 16) 32
_________________________________________________________________
dense_2 (Dense) (None, 32) 544
_________________________________________________________________
dense_3 (Dense) (None, 2) 66
=================================================================
Total params: 642
Trainable params: 642
Non-trainable params: 0
_________________________________________________________________
We can also inspect attributes about the model, like the optimizer and loss by calling model.optimizer
and model.loss
on the loaded model and compare the results to the previously
saved model.
This is the most encompassing way to save and load a model.
Saving and loading only the architecture of the model
There is another way we save only the architecture of the model. This will not save the model weights, configurations, optimizer, loss or anything else. This only saves the architecture of the model.
We can do this by calling model.to_json()
. This will save the architecture of the model as a JSON string. If we print out the string, we can see exactly what this looks like.
json_string = model.to_json() json_string
'{"class_name": "Sequential", "config": {"name": "sequential", "layers": [{"class_name": "Dense", "config": {"name":
"dense", "trainable": true, "batch_input_shape": [null, 1], "dtype": "float32", "units": 16, "activation": "relu", "use_bias": true,
"kernel_initializer": {"class_name": "GlorotUniform", "config": {"seed": null}}, "bias_initializer": {"class_name": "Zeros", "config": {}},
"kernel_regularizer": null, "bias_regularizer": null, "activity_regularizer": null, "kernel_constraint": null, "bias_constraint": null}},
{"class_name": "Dense", "config": {"name": "dense_1", "trainable": true, "dtype": "float32", "units": 32, "activation": "relu", "use_bias": true,
"kernel_initializer": {"class_name": "GlorotUniform", "config": {"seed": null}}, "bias_initializer": {"class_name": "Zeros", "config": {}},
"kernel_regularizer": null, "bias_regularizer": null, "activity_regularizer": null, "kernel_constraint": null, "bias_constraint": null}},
{"class_name": "Dense", "config": {"name": "dense_2", "trainable": true, "dtype": "float32", "units": 2, "activation": "softmax", "use_bias":
true, "kernel_initializer": {"class_name": "GlorotUniform", "config": {"seed": null}}, "bias_initializer": {"class_name": "Zeros", "config": {}},
"kernel_regularizer": null, "bias_regularizer": null, "activity_regularizer": null, "kernel_constraint": null, "bias_constraint": null}}]},
"keras_version": "2.2.4-tf", "backend": "tensorflow"}'
Now that we have this saved, we can create a new model from it. First we'll import the needed model_from_json
function, and then we can load the model architecture.
from tensorflow.keras.models import model_from_json
model_architecture = model_from_json(json_string)
By printing the summary of the model, we can verify that the new model has the same architecture of the model that was previously saved.
model_architecture.summary()
Model: "sequential"
_________________________________________________________________
Layer (type) Output Shape Param #
=================================================================
dense (Dense) (None, 16) 32
_________________________________________________________________
dense_1 (Dense) (None, 32) 544
_________________________________________________________________
dense_2 (Dense) (None, 2) 66
=================================================================
Total params: 642
Trainable params: 642
Non-trainable params: 0
_________________________________________________________________
Note, we can also use this same approach to saving and loading the model architecture to and from a YAML string. To do so, we use the functions to_yaml()
and model_from_yaml()
in
the same fashion as we called the json functions.
Saving and loading the weights of the model
The last saving mechanism we'll discuss only saves the weights of the model.
We can do this by calling model.save_weights()
and passing in the path and file name to save the weights to with an h5
extension.
model.save_weights('models/my_model_weights.h5')
At a later point, we could then load the saved weights in to a new model, but the new model will need to have the same architecture as the old model before the weights can be saved.
model2 = Sequential([
Dense(units=16, input_shape=(1,), activation='relu'),
Dense(units=32, activation='relu'),
Dense(units=2, activation='softmax')
])
model2.load_weights('models/my_model_weights.h5')
We've now seen how to save only the weights of a model and deploy those weights to a new model, how to save only the architecture and then deploy that architecture to a model, and how to save everything about a model and deploy it in its entirety at a later time. Each of these saving and loading mechanisms may come in useful in differing scenarios.
quiz
resources
updates
Committed by on