Build and Train a Convolutional Neural Network with TensorFlow's Keras API
text
Build and train a convolutional neural network with TensorFlow's Keras API
In this episode, we'll demonstrate how to build a simple convolutional neural network (CNN) and train it on images of cats and dogs using TensorFlow's Keras API.
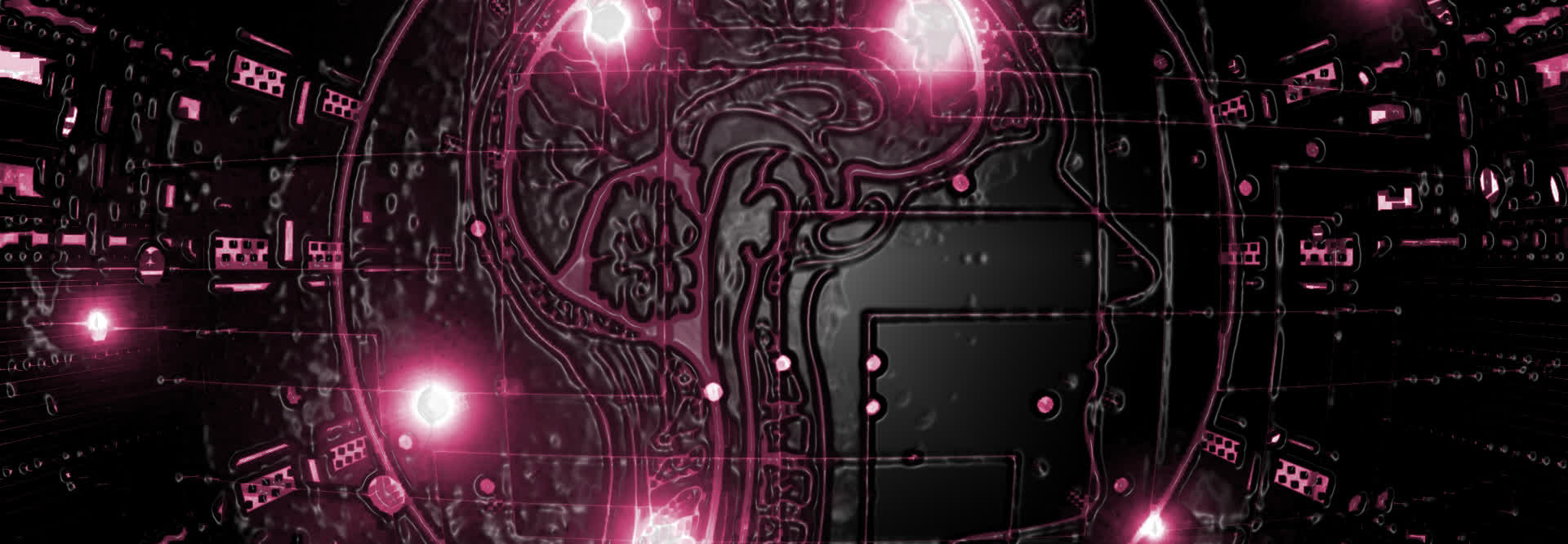
We'll be working with the image data we prepared in the last episode. Be sure that you have gone through that episode first to get and prepare the data, and also ensure that you still have all of the imports we brought in last time, as we'll be continuing to make use of them here.
Build a simple CNN
To build the CNN, we'll use a Keras Sequential
model. Recall, we first introduced a Sequential
model in an
earlier episode.
model = Sequential([
Conv2D(filters=32, kernel_size=(3, 3), activation='relu', padding = 'same', input_shape=(224,224,3)),
MaxPool2D(pool_size=(2, 2), strides=2),
Conv2D(filters=64, kernel_size=(3, 3), activation='relu', padding = 'same'),
MaxPool2D(pool_size=(2, 2), strides=2),
Flatten(),
Dense(units=2, activation='softmax')
])
The first layer in the model is a 2-dimensional convolutional layer. This layer will have 32
output filters each with a kernel size of 3x3
, and we'll use the
relu
activation function.
Note that the choice for the number of output filters specified is arbitrary, and the chosen kernel size of 3x3
is generally a very common size to use. You can experiment by choosing different
values for these parameters.
We enable
zero-padding by specifying padding = 'same'
.
On the first layer only, we also specify the input_shape
, which is the shape of our data. Our images are 224
pixels high and 224
pixels wide and have 3
color channels: RGB. This gives us an input_shape
of (224,224,3)
.
We then add a max pooling layer to pool and reduce the dimensionality of the data. Note, to gain a fundamental understanding of max pooling, zero padding, convolutional filters, and convolutional neural networks, check out the Deep Learning Fundamentals course.
We follow this by adding another convolutional layer with the exact specs as the earlier one, except for this second Conv2D
layer has 64
filters. The choice of 64
here
is again arbitrary, but the general choice of having more filters in later layers than in earlier ones is common. This layer is again followed by the same type of MaxPool2D
layer.
We then Flatten
the output from the convolutional layer and pass it to a Dense
layer. This Dense
layer is the output layer of the network, and so it has
2
nodes, one for cat and one for dog. We'll use the softmax
activation function on our output so that the output for each sample is a probability distribution over the outputs
of cat and dog.
We can check out a summary of the model by calling model.summary()
.
Model: "sequential"
_________________________________________________________________
Layer (type) Output Shape Param #
=================================================================
conv2d (Conv2D) (None, 224, 224, 32) 896
_________________________________________________________________
max_pooling2d (MaxPooling2D) (None, 112, 112, 32) 0
_________________________________________________________________
conv2d_1 (Conv2D) (None, 112, 112, 64) 18496
_________________________________________________________________
max_pooling2d_1 (MaxPooling2 (None, 56, 56, 64) 0
_________________________________________________________________
flatten (Flatten) (None, 200704) 0
_________________________________________________________________
dense (Dense) (None, 2) 401410
=================================================================
Total params: 420,802
Trainable params: 420,802
Non-trainable params: 0
_________________________________________________________________
Now that the model is built, we compile
the model using the Adam
optimizer with a learning rate of 0.0001
, a loss of categorical_cross_entropy
, and we'll
look at accuracy
as our performance metric
. Again, if you need a fundamental understanding of any of these topics, check out the
Deep Learning Fundamentals course.
model.compile(optimizer=Adam(learning_rate=0.0001), loss='categorical_crossentropy', metrics=['accuracy'])
Note that when we have only two classes, we could instead configure our output layer to have only one output, rather than two, and use binary_crossentropy
as our loss, rather than categorical_crossentropy
.
Both options work equally well and achieve the exact same result.
With binary_crossentropy
, however, the last layer would need to use sigmoid
, rather than softmax
, as its activation function.
Train a simple CNN
Now it's time to train the model.
We've already introduced the model.fit()
function to train a model in a
previous episode. We'll be using it in the same fashion here, except for now, we'll be passing in our newly introduced DirectoryIterators
train_batches
and valid_batches
to train and validate the model. Recall, these were created in the
last episode.
model.fit(x=train_batches,
steps_per_epoch=len(train_batches),
validation_data=valid_batches,
validation_steps=len(valid_batches),
epochs=10,
verbose=2
)
We need to specify steps_per_epoch
to indicate how many batches of samples from our training set should be passed to the model before declaring one epoch complete. Since we have 1000
samples in our training set, and our batch size is 10
, then we set steps_per_epoch
to be 100
, since 100
batches of 10
samples each will encompass our entire training set.
We're able to use len(train_batches)
as a more general way to specify this value, as the length of train_batches
is equal to 100
since it is made up of 100
batches of 10
samples. Similarly, we specify validation_steps
in the same fashion but with using valid_batches
.
We're specifying 10
as the number of epochs
we'd like to run, and setting the verbose
parameter to 2
, which just specifies the verbosity
of the log output printed to the console during training.
When we run this line of code, we can see the output of the model over 10
epochs.
Epoch 1/10
Train for 100 steps, validate for 20 steps
Epoch 1/10
100/100 - 6s - loss: 14.5537 - accuracy: 0.5470 - val_loss: 4.7720 - val_accuracy: 0.6150
Epoch 2/10
100/100 - 3s - loss: 2.1476 - accuracy: 0.7520 - val_loss: 2.5369 - val_accuracy: 0.6600
Epoch 3/10
100/100 - 3s - loss: 0.5725 - accuracy: 0.8840 - val_loss: 2.7590 - val_accuracy: 0.5950
Epoch 4/10
100/100 - 3s - loss: 0.1467 - accuracy: 0.9460 - val_loss: 2.3967 - val_accuracy: 0.6300
Epoch 5/10
100/100 - 3s - loss: 0.0291 - accuracy: 0.9880 - val_loss: 2.1665 - val_accuracy: 0.6550
Epoch 6/10
100/100 - 3s - loss: 0.0039 - accuracy: 1.0000 - val_loss: 2.0959 - val_accuracy: 0.6600
Epoch 7/10
100/100 - 3s - loss: 0.0019 - accuracy: 1.0000 - val_loss: 2.0650 - val_accuracy: 0.6800
Epoch 8/10
100/100 - 3s - loss: 0.0014 - accuracy: 1.0000 - val_loss: 2.0739 - val_accuracy: 0.6750
Epoch 9/10
100/100 - 3s - loss: 0.0010 - accuracy: 1.0000 - val_loss: 2.0598 - val_accuracy: 0.6850
Epoch 10/10
100/100 - 3s - loss: 8.4486e-04 - accuracy: 1.0000 - val_loss: 2.0595 - val_accuracy: 0.6850
From this output, we can see the performance of this simple model on the training set is great, with accuracy reaching 100%
and loss nearing 0
, however, by comparing these results
to the validation metrics, we can see that our model is vastly
overfitting to the training data.
At this point, we could continue to work on this model to combat overfitting, or we could try another approach of using a pre-trained model on this data. We'll explore the latter in the upcoming episodes!
quiz
resources
updates
Committed by on