Predict with a Fine-Tuned Neural Network with TensorFlow's Keras API
text
Predict with a fine-tuned neural network with TensorFlow's Keras API
In this episode, we'll demonstrate how to use the fine-tuned VGG16 model that we trained in the last episode to predict on images of cats and dogs in our test set.
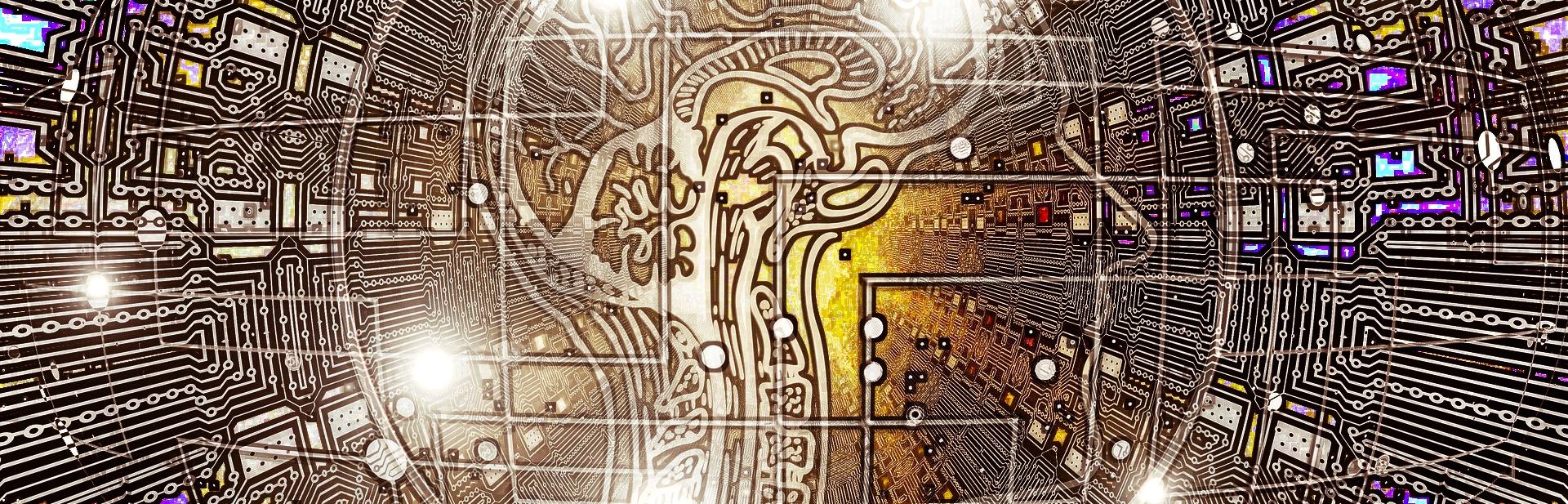
First, ensure that you have all the code in place from the last episode because we'll be picking up directly where we left off there and will be using several variables and imports that were already defined and brought in previously.
Picking up with the code, we'll first get a batch of test samples and their corresponding labels from the test set, and plot them to see what the data looks like.
test_imgs, test_labels = next(test_batches)
plotImages(test_imgs)
print(test_labels)

Recall that this is the same test set we used in a previous episode to test the model we built from scratch, and the color in the images appears to be distorted due to the VGG16 preprocessing we discussed previously.
We now call model.predict
to have the model predict on the test data.
predictions = model.predict(x=test_batches, steps=len(test_batches), verbose=0)
We pass in the test set, test_batches
, and set steps
to be then length of test_batches
. Similar to steps_per_epoch
that we specified in the last episode, steps
specifies how many batches to yield from the test set before declaring one prediction round complete.
Plot predictions with a confusion matrix
We're now going to create a confusion matrix so we can visualize our predictions. The code we'll use to do this is exactly the same as we used in a previous episode, so again, be sure to check that one out if you need a refresher explanation.
cm = confusion_matrix(y_true=test_batches.classes, y_pred=np.argmax(predictions, axis=-1))
cm_plot_labels = ['cat','dog']
plot_confusion_matrix(cm=cm, classes=cm_plot_labels, title='Confusion Matrix')
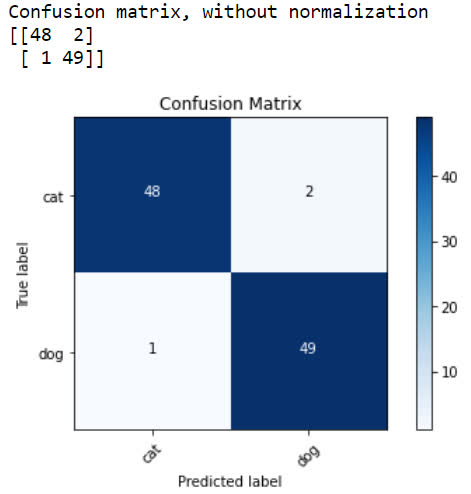
We can see that the model incorrectly predicted only 3
samples out of 100
. This gives us 97%
accuracy on the test set, proving this model to be much more capable of
generalizing than the previous CNN we built from scratch.
quiz
resources
updates
Committed by on