Process Images for Fine-Tuned MobileNet with TensorFlow's Keras API
text
Process Images for Fine-Tuned MobileNet with TensorFlow's Keras API
In this episode, we'll be building on what we've learned about MobileNet to prepare and process our own custom image data set of sign language digits. These images will be later used to train our fine-tuned MobileNet model.
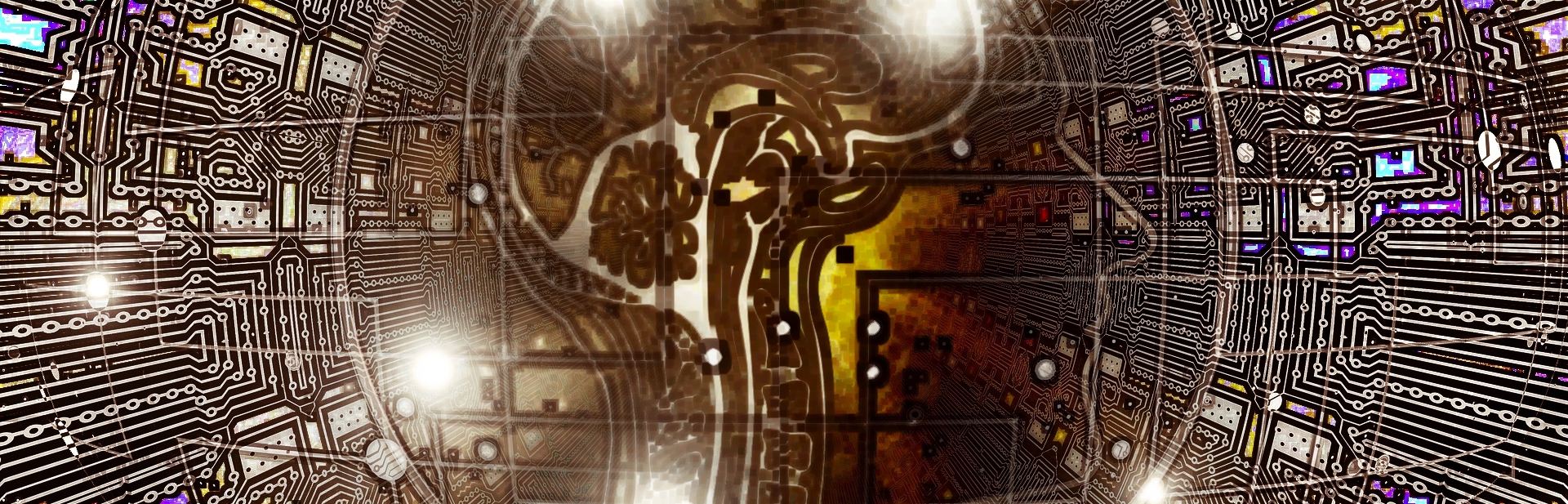
Obtain the data
The new data set we'll be working with now does not have classes that were included in ImageNet, nor very similar to the ImageNet classes that MobileNet was originally trained on. We'll later go through the process together for tuning the model to predict on this new data.
This data set contains images of sign language digits. There are ten classes, labeled as 0
through 9
, and each class is made up of images of hands showing the sign for that particular
digit.
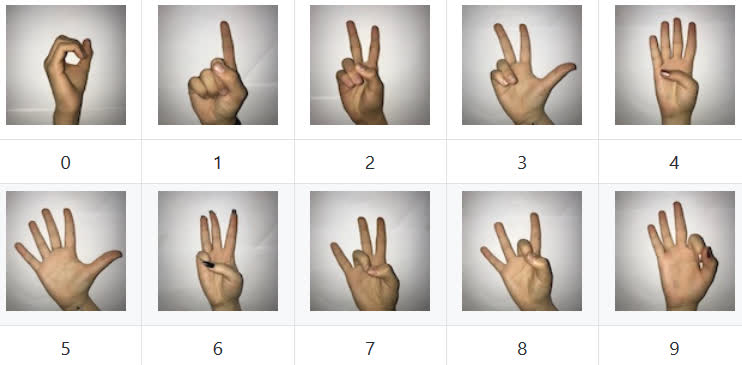
Each class has between 204
and 208
samples. The total data set contains 2062
samples.
This data set is available as grayscale images on Kaggle and is also available as RGB images on Github. We'll be using the RGB data set.
Organize the data
Before we can begin tuning the model, we first need to organize our images on disk. They will be organized in the same fashion as the cat and dog data set we used in previous episodes.
With code, we'll create the directories for the train, validation, and test sets, organize the images into their respective classes of 0
through 9
on disk, and then shuffle
the data set and split the images into train, validation, and test sets.
First, download the data and save to your-current-nb-directory/data/Sign-Language-Digits-Dataset
. Extract sub-directories labeled 0
through 9
and nest these directories
directly underneath the Sign-Language-Digits-Dataset
directory.
Before using the script below to organize the data, be sure you have the imports brought in from earlier.
Note, the first two lines of code change directory into the relevant directory where the data is stored and then checks to ensure that the directory structure hasn't already been set up.
# Organize data into train, valid, test dirs
os.chdir('data/Sign-Language-Digits-Dataset')
if os.path.isdir('train/0/') is False:
os.mkdir('train')
os.mkdir('valid')
os.mkdir('test')
for i in range(0, 10):
shutil.move(f'{i}', 'train')
os.mkdir(f'valid/{i}')
os.mkdir(f'test/{i}')
valid_samples = random.sample(os.listdir(f'train/{i}'), 30)
for j in valid_samples:
shutil.move(f'train/{i}/{j}', f'valid/{i}')
test_samples = random.sample(os.listdir(f'train/{i}'), 5)
for k in test_samples:
shutil.move(f'train/{i}/{k}', f'test/{i}')
os.chdir('../..')
First, we create train
, valid
, and test
directories.
We then move all class directories (0
- 9
) with their respective images from Sign-Language-Digits-Dataset
into the train
directory.
We then make class directories (0
- 9
) for the valid
and test
data sets as well.
We then loop through each class directory in the train
directory and randomly move 30
images from each class into the corresponding class directory in valid
and
5
images from each class into the corresponding class directory in test
.
Finally, we end by moving back into current notebook directory.
Process the data
After our image data is all organized on disk, we need to create the directory iterators for the train, validation, and test sets in the exact same way as we did for the cat and dog data set that we previously used.
train_path = 'data/Sign-Language-Digits-Dataset/train'
valid_path = 'data/Sign-Language-Digits-Dataset/valid'
test_path = 'data/Sign-Language-Digits-Dataset/test'
train_batches = ImageDataGenerator(preprocessing_function=tf.keras.applications.mobilenet.preprocess_input).flow_from_directory(
directory=train_path, target_size=(224,224), batch_size=10)
valid_batches = ImageDataGenerator(preprocessing_function=tf.keras.applications.mobilenet.preprocess_input).flow_from_directory(
directory=valid_path, target_size=(224,224), batch_size=10)
test_batches = ImageDataGenerator(preprocessing_function=tf.keras.applications.mobilenet.preprocess_input).flow_from_directory(
directory=test_path, target_size=(224,224), batch_size=10, shuffle=False)
Notice the preprocessing_function
parameter we're supplying to ImageDataGenerator
. We're setting this equal to tf.keras.applications.mobilenet.preprocess_input
.
This is going to do the necessary MobileNet preprocessing on the images (explained
previously) obtained from flow_from_directory()
.
To flow_from directory()
, we're passing in the path to the data set, the target_size
to resize the images to, and the batch_size
we're choosing to use
for training. We do this exact same thing for all three data sets: train, validation, and test.
For test_batches
, we're also supplying one additional parameter, shuffle=False
, which causes the test dataset to not be shuffled, so that we can access the corresponding
non-shuffled test labels to plot to a confusion matrix later.
The data has now been prepared and processed. Next, we'll move on to fine-tuning the model.
quiz
resources
updates
Committed by on