TensorFlow.js - Convert Keras model to Layers API format
text
Client-side Neural Networks
What's up, guys? In this post, we'll continue getting acquainted with the idea of client-side neural networks, and we'll kick things off by seeing how we can use TensorFlow's model converter tool to convert Keras models into TensorFlow.js models.
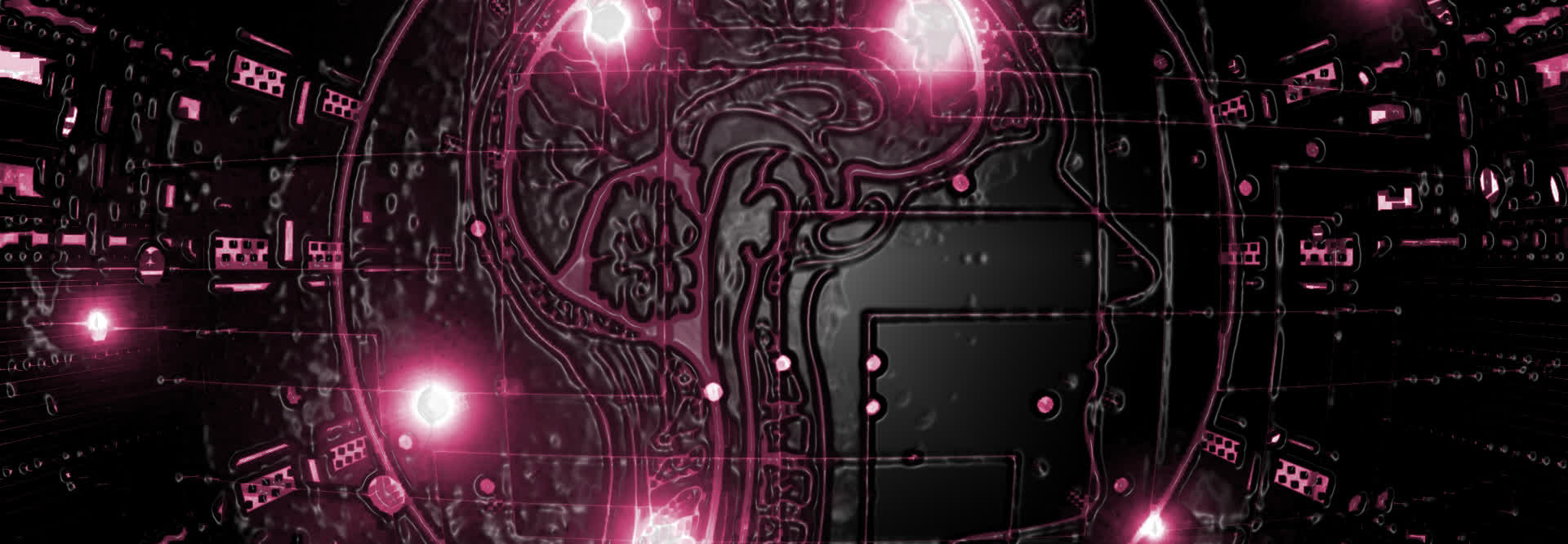
This will allow us to take models that have already been built and trained with Keras and make use of them in the browser with TensorFlow.js, so let's get to it.
TensorFlow.js has what they call the Layers API, which is a high-level neural network API inspired by Keras, and we'll see that what we can do with this API and how we use it is super similar to what we've historically been able to do with Keras.
Given this, it makes sense that we should be able to take a model that we built in Keras, or that we trained in Keras and port it over to TensorFlow.js and use it in the browser with the Layers API, right?
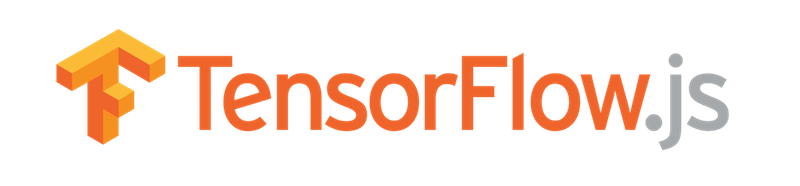
Otherwise, the alternative would be to build a model from scratch and train it from scratch in the browser, and as we discussed in the last video, that's not always going to be ideal. So, having the ability and the convenience to convert a pre-built or pre-trained Keras model to run in the browser is definitely going to come in handy.
How can we do this?
Installing the Tensorflow.js model converter tool
First, we need to install the TensorFlow.js model converter tool. From a Python environment, probably one where Keras is already installed, we run
pip install tensorflowjs
from the terminal. Once we have this, we can convert a Keras model into a TensorFlow.js model.
pip install tensorflowjs
There are two methods for doing the conversion, and we'll demo both.
Conversion method 1
The first way is making use of the converter through the terminal or command line.
We'd want to use this method for Keras models that we've already saved to disk as an
h5
file. Recall from an earlier video that covered saving and loading Keras models, we have multiple ways we can save a model or save parts of a model, like just the weights, or just the architecture.
To convert a Keras model into a TensorFlow.js model though, we need to have saved the entire model with the weights, the architecture, everything in an
h5
file. Currently that's done using the Keras
model.save()
function.
I already have a sample model we built in an earlier Keras video that I've saved to disk.
Two example models can be downloaded from Keras here:
I'm in the terminal now where we'll run the
tensorflowjs_converter
program.
tensorflowjs_converter --input_format keras medical_trial_model.h5 SimpleModel/
We run
tensorflowjs_converter
and specify what kind of input the converter should expect, so we supply
--input_format keras
. Then we supply the path to the saved
h5
file and the path to the output directory where we want our converted model to be placed.
The output directory needs to be a directory that's solely for holding the converted model. There will be multiple files, so don't just specify your desktop or something like that.
When we run this, we get this warning regarding deprecation, but it's not hurting us for anything we're doing here. And that's it for the first method! We'll see in a few moments the format of the converted model, but before we do that...
Conversion method 2
This is going to be done directly using Python, and this method is for when we're working with a Keras model and we want to go ahead and convert it on-the-spot to a TensorFlow.js model without necessarily needing to save it to an
h5
file first.
We're in a Jupyter notebook where we're importing Keras and the TensorFlow.js library. I'm going to demo this with the VGG16 model because we'll be making use of this one in a future video anyway, but this conversion will work for any model you build with Keras.
import tensorflow as tf
from tensorflow import keras
import tensorflowjs as tfjs
vgg16 = tf.keras.applications.vgg16.VGG16()
tfjs.converters.save_keras_model(vgg16,'../../tfjs/tfjs-models/VGG16')
We have this vgg16
model that's created by calling
keras.applications.vgg16.VGG16()
, and then we call
tensorflowjs.converters.save_keras_model()
. To this function, we supply the model that we're converting as well as the path to the output directory where we want the converted TensorFlow.js
model to be placed. And that's it for the second method!
For preparedness, you should also do the same for the MobileNet model, as we'll be using it in a future episode as well.
Output directories
Let's check out what the output from these conversions looks like!
We're going to look at the smaller model that we first converted from the terminal.
./SimpleModel group1-shard1of1 group2-shard1of1 group3-shard1of1 model.json
We're inside of this directory called
SimpleModel
, which is the output directory we specified whenever we converted the first model, and we have a few files here. We have this one file called
model.json
, which contains the model architecture and metadata for the weight files. The corresponding weight files are these sharded files that contain all the weights from the model and are
stored in binary format.
The larger and more complex the model is, the more weight files there will be. This model was small with only a couple dense layers and about 640
learnable parameters, but the VGG16 model we
converted, on the other hand, with over 140
million learnable parameters has 144
corresponding weight files.
Wrapping up
Alright, that's how we can convert our existing Keras models into TensorFlow.js models!
We'll see how these models and their corresponding weights are loaded in the browser in a future post when we start building our browser application to run these models. Let me know in the comments if you're ready to start building, and I'll see ya in the next one!
quiz
resources
updates
Committed by on