Learnable parameters ("trainable params") in a Keras model
text
Trainable parameters in a Keras model
In this episode, we'll discuss how we can quickly access and calculate the number of learnable parameters in a Keras model. Let's get to it!
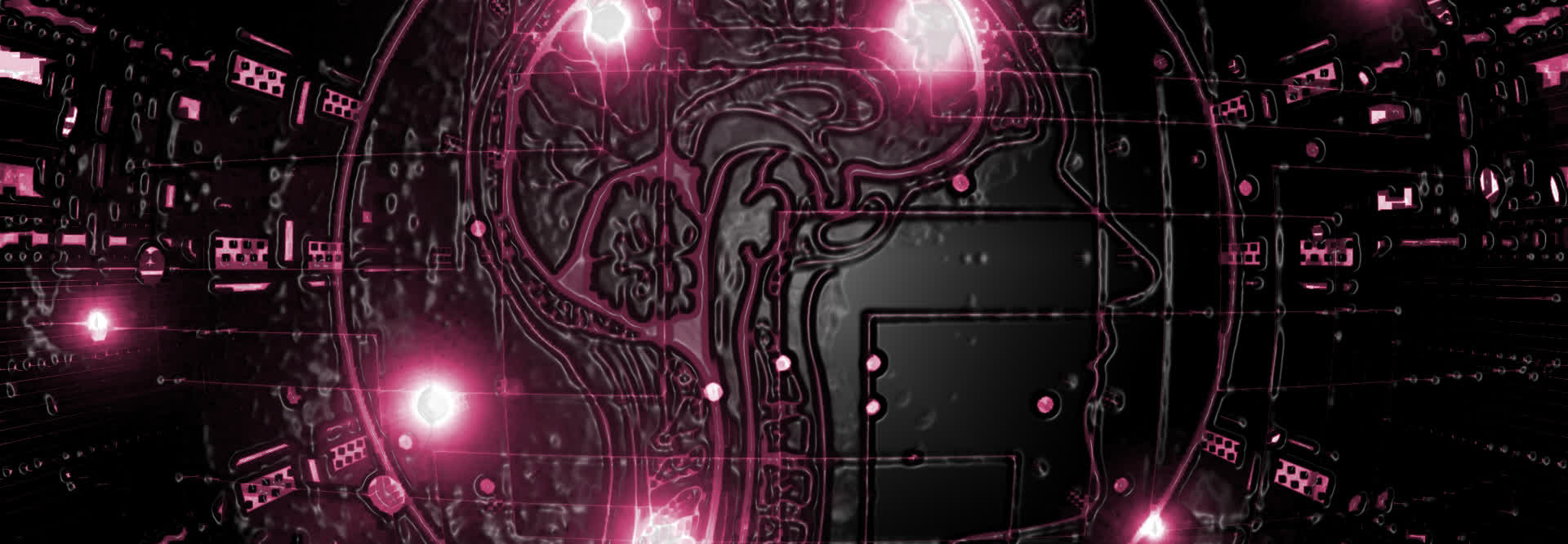
Keras model example
Here, we have a very basic Keras Sequential
model, which consists of an input layer with 2
input features, or 2
nodes, a single hidden layer with 3
nodes,
and an output layer with 2
nodes. You should already be familiar with this type of model based on of earlier episodes in this series.
from keras.models import Sequential
from keras.layers import Dense, Activation
model = Sequential([
Dense(3, input_shape=(2,), activation='relu'),
Dense(2, activation='softmax')
])
Previously, we've utilized the model.summary()
function to check out the architecture of our model, or to verify the output shape from each layer when we learned about
zero-padding, for example, but we never talked about the last column called Param #
. This column shows us the number of learnable parameters within each layer.
model.summary()
Layer (type) | Output Shape | Param # |
---|---|---|
dense_3 (Dense) |
(None, 3) |
9 |
dense_4 (Dense) |
(None, 2) |
8 |
Total params: 17
Trainable params: 17
Non-trainable params: 0
At the bottom of the summary, we have the total number of learnable parameters within the network displayed, which Keras refers to as Trainable params
.
We've already discussed what a learnable parameter is and how to calculate the number of these parameters in each layer and within the entire model over in the deep learning fundamentals series, so go check that out if you're not sure what these things are, and then head back over here.
This model is actually an exact implementation of the conceptual model we worked with over in that previous episode. If you recall, in our single hidden layer, we indeed calculated that there were 9
learnable parameters, just as Keras is showing us in this output. That was from the 6
weights and the 3
biases in this layer.
We also calculated that the output layer contained 8
learnable parameters consisting of 6
weights, and 2
biases.
Now, recall we also previously showed how we can access the weights and biases within the model by calling the get_weights()
function that we discussed in our video on
bias initialization.
By calling this function, we can view how the number of weights and biases we calculated in each layer add up to the totals we get in the param
column of model.summary()
.
model.get_weights()
[array([[0.628, 0.578, 0.374],
[-0.881, 0.839, -1.035]], dtype=float32),
array([0., 0., 0.], dtype=float32),
array([[-0.557, -0.206],
[0.701, 0.786],
[-0.074, 0.799]], dtype=float32),
array([0., 0.], dtype=float32)]
So here, we first have our weights for the hidden layer, and recall, these are randomly initialized using Xavier or Glorot initialization by default in Keras. So we have these 6
random numbers
here corresponding to the 6
weights we calculated for this layer.
Then, we have our 3
bias terms, which we previously learned were initialized to zeros by default. Note that the the sum of these two numbers does indeed add up to the 9
learnable
parameters that was given for this layer in the output of model.summary()
.
We can also do the same thing for our output layer. So again, we have 6
weights that have been randomly initialized, and we have 2
bias terms initialized to zeros. Summing these
two numbers, we have 8
learnable parameters, again matching the output for this layer in model.summary()
.
Adding 8
to 9
, we have 17
total learnable parameters, which corresponds exactly to what Keras shows us for the total number of trainable params
in the output
above.
So there you have it. That's how we can access and confirm the total number of learnable parameters in a Keras model. Next, we'll be discussing how this is done with a convolutional neural network as well, and we'll see there are just some slight differences in the calculations we have to consider when dealing with CNNs, so stay tuned for that!
quiz
resources
updates
Committed by on