Backpropagation explained | Part 3 - Mathematical observations
text
Mathematical observations for backpropagation
Hey, what's going on everyone? In this post, we're going to make mathematical observations about some facts we already know about the training process of an artificial neural network.
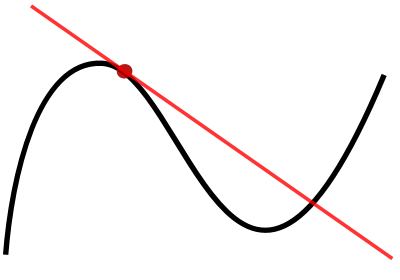
We'll then be using these observations going forward in our calculations for backpropagation. Let's get to it.
The path forward
In our last post, we focused on the mathematical notation and definitions that we would be using going forward to show how backpropagation mathematically works to calculate the gradient of the loss function.
Well, now's the time that we'll start making use of them, so it's crucial that you have a full understanding of everything we covered in that post first.
Here, we're going to be making some mathematical observations about the training process of a neural network. The observations we'll be making are actually facts that we already know conceptually, but we'll now just be expressing them mathematically.
We'll be making these observations because the math for backprop that comes next, particularly, the differentiation of the loss function with respect to the weights, is going to make use of these observations.
We're first going to start out by making an observation regarding how we can mathematically express the loss function. We're then going to make observations around how we express the input and the output for any given node mathematically.
Lastly, we'll observe what method we'll be using to differentiate the loss function via backpropagation. Alright, let's begin.
Loss \(C_{0}\)
Observe that the expression
is the squared difference of the activation output and the desired output for node \(j\) in the output layer \(L\). This can be interpreted as the loss for node \(j\) in layer \(L\).
Therefore, to calculate the total loss, we should sum this squared difference for each node \(j\) in the output layer \(L\).
This is expressed as
Input \(z_{j}^{(l)}\)
We know that the input for node \(j\) in layer \(l\) is the weighted sum of the activation outputs from the previous layer \(l-1\).
An individual term from the sum looks like this:
So, the input for a given node \(j\) in layer \(l\) is expressed as
Activation Output \(a_{j}^{(l)}\)
We know that the activation output of a given node \(j\) in layer \(l\) is the result of passing the input, \(z_{j}^{\left( l\right) }\), to whatever activation function we choose to use \(g^{\left( l\right) }\).
Therefore, the activation output of node \(j\) in layer \(l\) is expressed as
\(C_{0}\) as a composition of functions
Recall the definition of \(C_{0}\),
So the loss of a single node \(j\) in the output layer \(L\) can be expressed as
We see that \(C_{0_{j}}\) is a function of the activation output of node \(j\) in layer \(L\), and so we can express \(C_{0_{j}}\) as a function of \(a_{j}^{\left( L\right) }\) as
Observe from the definition of \(C_{0_{j}}\) that \(C_{0_{j}}\) also depends on \( y_{j}\). Since \(y_{j}\) is a constant, we only observe \(C_{0_{j}}\) as a function of \(a_{j}^{\left( L\right) }\), and \(y_{j}\) as a parameter that helps define this function.
The activation output of node \(j\) in the output layer \(L\) is a function of the input for node \(j\). From an earlier observation, we know we can express this as
The input for node \(j\) is a function of all the weights connected to node \(j\). We can express \(z_{j}^{\left( L\right) }\) as a function of \(w_{j}^{\left( L\right) }\) as
Therefore,
Given this, we can see that \(C_{0}\) is a composition of functions.
We know that
and so using the same logic, we observe that the total loss of the network for a single input is also a composition of functions. This is useful in order to understand how to differentiate \(C_{0}\).

To differentiate a composition of functions, we use the chain rule.
Wrapping up
Alright, so now we should understand the ways we can mathematically express the loss function of a neural network, as well as the input and the activation output of any given node.
Additionally, it should be clear now that the loss function is actually a composition of functions, and so to differentiate the loss with respect to the weights in the network, we'll need to use the chain rule.
Going forward, we'll be using the observations that we learned here in the relatively heavier math that we'll be using with backprop.
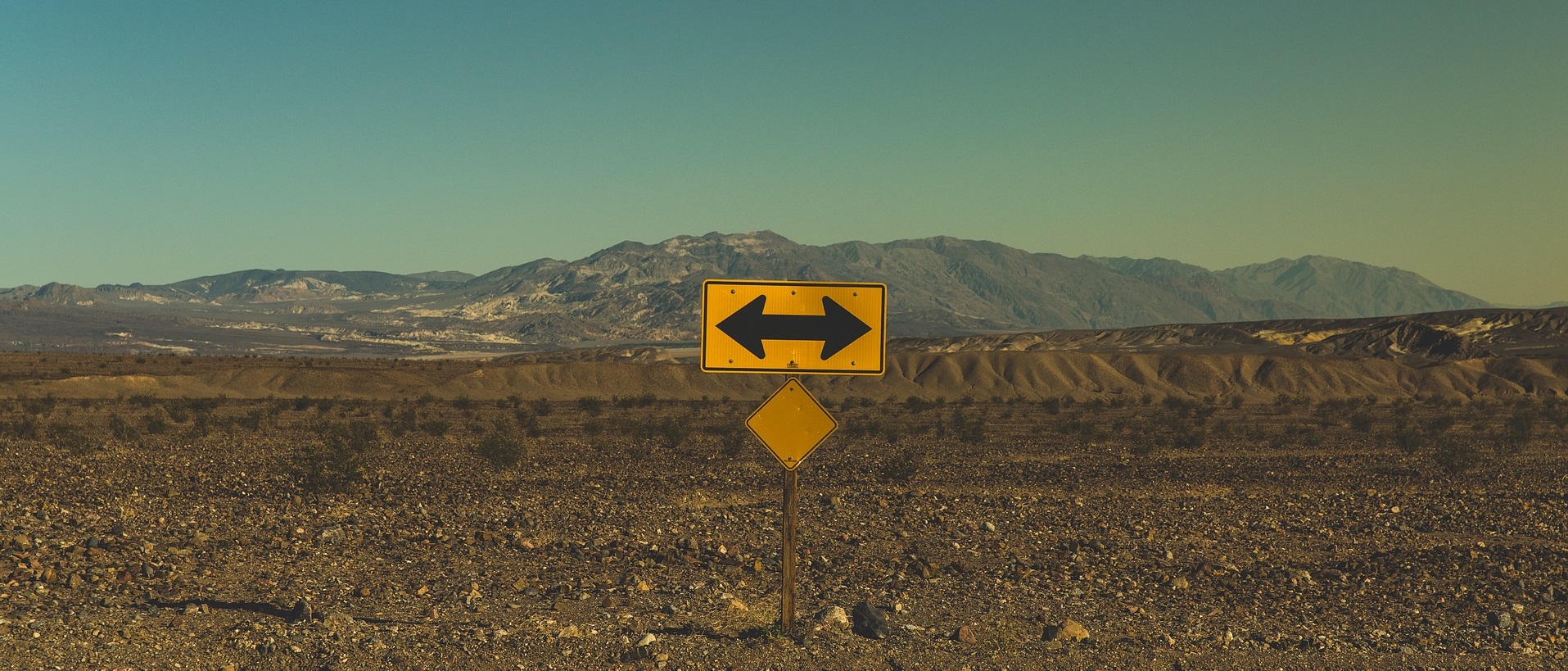
In the next post, we'll start getting exposure to this math. Before moving on to that though, take the time to make sure you understand these observations that we covered in this post and why we'll be working with the chain rule to differentiate the loss function. See ya next time!
quiz
resources
updates
Committed by on