Backpropagation explained | Part 4 - Calculating the gradient
text
Backpropagation explained | Part 4 - Calculating the gradient
Hey, what's going on everyone? In this episode, we're finally going to see how backpropagation calculates the gradient of the loss function with respect to the weights in a neural network.
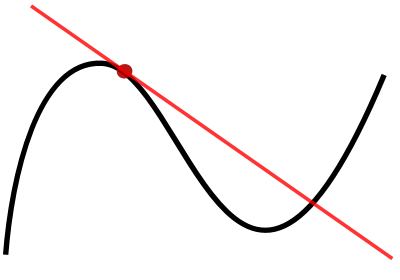
So let's get to it.
Our Task
We're now on episode number four in our journey through understanding backpropagation. In the last episode, we focused on how we can mathematically express certain facts about the training process.
Now we're going to be using these expressions to help us differentiate the loss of the neural network with respect to the weights.
Recall from the episode that covered the intuition for backpropagation that for stochastic gradient descent to update the weights of the network, it first needs to calculate the gradient of the loss with respect to these weights.
Calculating this gradient is exactly what we'll be focusing on in this episode.
We're first going to start out by checking out the equation that backprop uses to differentiate the loss with respect to weights in the network.
Then, we'll see that this equation is made up of multiple terms. This will allow us to break down and focus on each of these terms individually.
Lastly, we'll take the results from each term and combine them to obtain the final result, which will be the gradient of the loss function.
Alright, let's begin.
Derivative of the Loss Function with Respect to the Weights
Let's look at a single weight that connects node
This weight is denoted as
The derivative of the loss
Since
This is expressed as
Let's break down each term from the expression on the right hand side of the above equation.
The first term:
We know that
Therefore,
Expanding the sum, we see
Observe that the loss from the network for a single input sample will respond to a small change in the activation output from node
The second term:
We know that for each node
and since
Therefore,
Therefore, this is just the direct derivative of
The third term:
We know that, for each node
Since
Therefore,
Expanding the sum, we see
The input for node
Combining terms
Combining all terms, we have
We Conclude
We've seen how to calculate the derivative of the loss with respect to one individual weight for one individual training sample.
To calculate the derivative of the loss with respect to this same particular weight,
This can be expressed as
We would then do this same process for each weight in the network to calculate the derivative of
Wrapping Up
At this point, we should now understand mathematically how backpropagation calculates the gradient of the loss with respect to the weights in the network.
We should also have a solid grip on all of the intermediate steps needed to do this calculation, and we should now be able to generalize the result we obtained for a single weight and a single sample to all the weights in the network for all training samples.
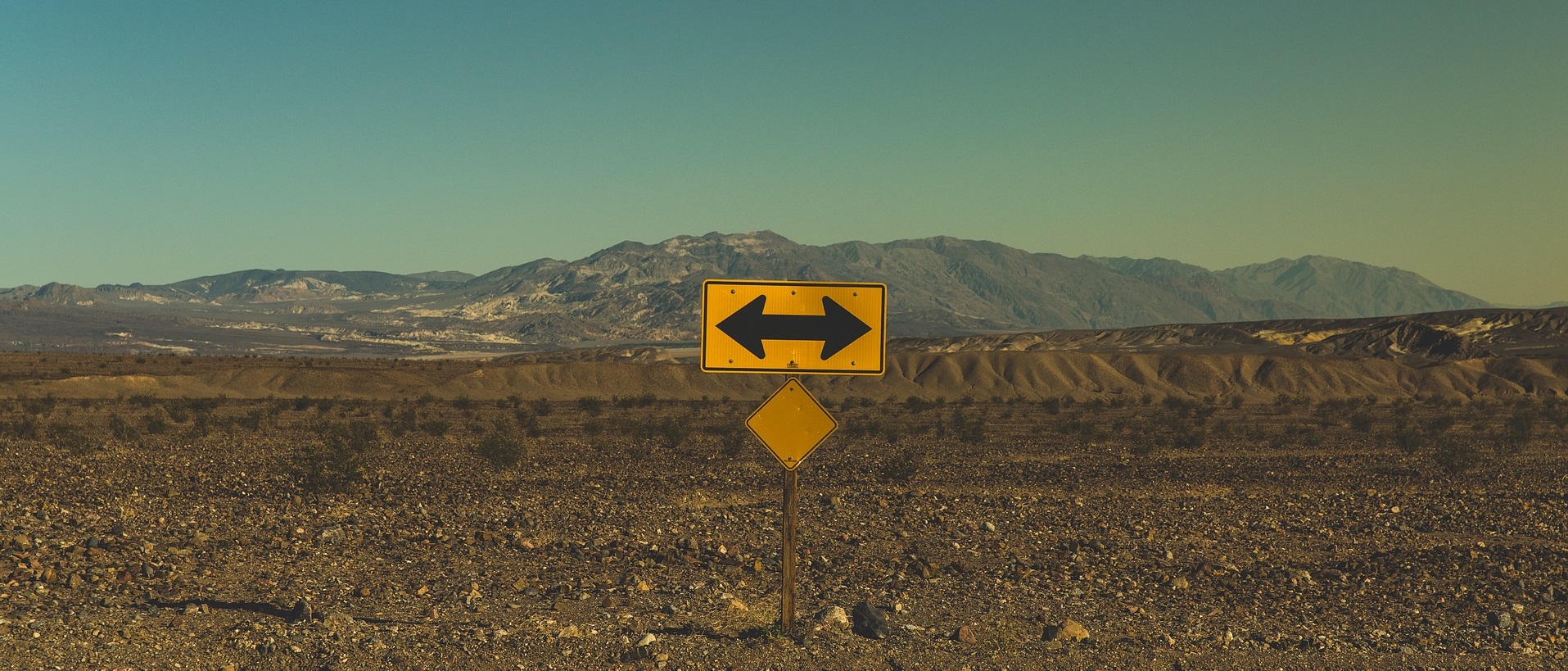
Now, we still haven't hit the point completely home by discussing the math that underlies the backwards movement of backpropagation that we discussed whenever we covered the intuition for backpropagation. Don't worry, we'll be doing that in the next episode. Thanks for reading. See you next time.
quiz
resources
updates
Committed by on
e32aca8
Committed by March 6, 2018
on