Training a Neural Network explained
text
Training an artificial neural network
In this post, we'll discuss what it means to train an artificial neural network. In a previous post, we went over the basic architecture of a general artificial neural network. Now, after configuring the architecture of the model, the next step is to train it.
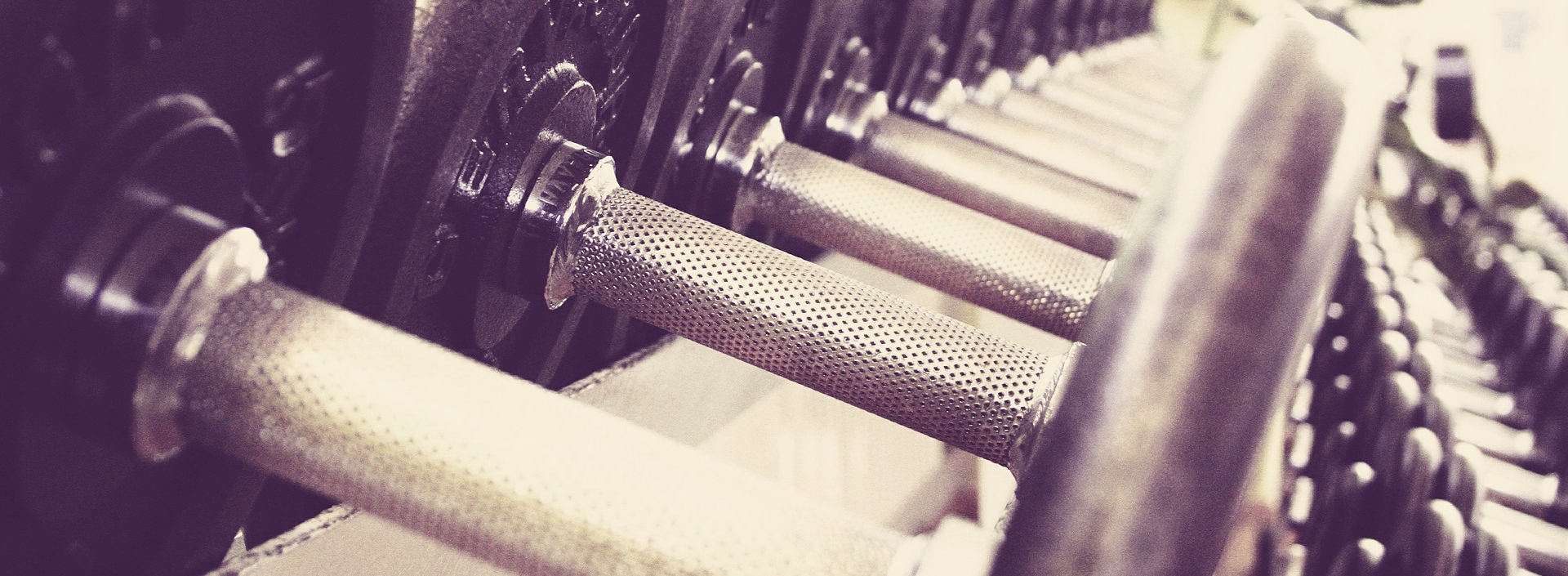
What is training?
When we train a model, we're basically trying to solve an optimization problem. We're trying to optimize the weights within the model. Our task is to find the weights that most accurately map our input data to the correct output class. This mapping is what the network must learn.
Recall, we touched on this idea in our post about layers. There, we showed how each connection between nodes has an arbitrary weight assigned to it. During training, these weights are iteratively updated and moved towards their optimal values.
// pseudocode
def train(model):
model.weights.update()
Optimization algorithm
The weights are optimized using what we call an optimization algorithm. The optimization process depends on the chosen optimization algorithm. We also use the term optimizer to refer to the chosen algorithm. The most widely known optimizer is called stochastic gradient descent, or more simply, SGD.
When we have any optimization problem, we must have an optimization objective, so now let's consider what SGD's objective is in optimizing the model's weights.
The objective of SGD is to minimize some given function that we call a loss function. So, SGD updates the model's weights in such a way as to make this loss function as close to its minimum value as possible.
Loss function
One common loss function is mean squared error (MSE), but there are several loss functions that we could use in its place. As deep learning practitioners, it's our job to decide which loss function to use. For now, let's just think of general loss functions, and later we'll look at specific loss functions in more detail.
Alright, but what is the actual loss we're talking about? Well, during training, we supply our model with data and the corresponding labels to that data.
For example, suppose we have a model that we want to train to classify whether images are either images of cats or images of dogs. We will supply our model with images of cats and dogs along with the labels for these images that state whether each image is of a cat or of a dog.
Suppose we give one image of a cat to our model. Once the forward pass is complete and the cat image data has flowed through the network, the model is going to provide an output at the end. This will consist of what the model thinks the image is, either a cat or a dog.
In a literal sense, the output will consist of probabilities for cat or dog. For example, it may assign a 75% probability to the image being a cat, and a 25% probability to it being a dog. In this case, the model is assigning a higher likelihood to the image being of a cat than of a dog.
- 75% chance it's a cat
- 25% chance it's a dog
If we stop and think about it for a moment, this is very similar to how humans make decisions. Everything is a prediction!
The loss is the error or difference between what the network is predicting for the image versus the true label of the image, and SGD will to try to minimize this error to make our model as accurate as possible in its predictions.
After passing all of our data through our model, we're going to continue passing the same data over and over again. This process of repeatedly sending the same data through the network is considered training. During this training process is when the model will actually learn. More about learning in the next post. So, through this process that's occurring with SGD iteratively, the model is able to learn from the data.
Conclusion
We know now generally what is happening during one forward pass of the data through the network. In the next post, we'll see how the model learns through multiple forward passes of the data and what exactly SGD is doing to minimize the loss function.
One thing to mention about this post is that we generally covered some new concepts, like the optimizer, loss, and a couple others. We'll definitely be diving into these in more detail, so stay tuned!
Hopefully now you have a general understanding about what it means to train a model. Check out the next post where we'll learn what's happening behind the scenes of this training and how the model learns during this process. See ya in the next one!
quiz
resources
updates
Committed by on