Batch Size in a Neural Network explained
text
Batch size in artificial neural networks
In this post, we'll discuss what it means to specify a batch size as it pertains to training an artificial neural network, and we'll also see how to specify the batch size for our model in code using Keras.
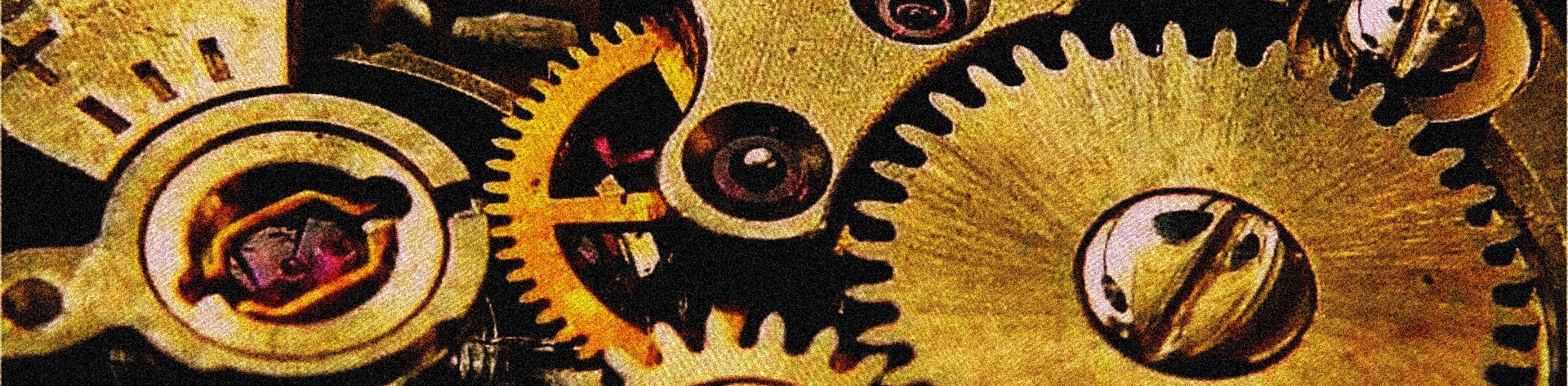
In our previous post on how an artificial neural network learns, we saw that when we train our model, we have to specify a batch size. Let's go ahead and discuss the details about this now.
Introducing batch size
Put simply, the batch size is the number of samples that will be passed through to the network at one time. Note that a batch is also commonly referred to as a mini-batch.
Now, recall that an epoch is one single pass over the entire training set to the network. The batch size and an epoch are not the same thing. Let's illustrate this with an example.
Batches in an epoch
Let's say we have 1000
images of dogs that we want to train our network on in order to identify different breeds of dogs. Now, let's say we specify our batch size to be
10
. This means that 10
images of dogs will be passed as a group, or as a batch, at one time to the network.
Given that a single epoch is one single pass of all the data through the network, it will take 100
batches to make up full epoch. We have 1000
images divided by a batch size of
10
, which equals 100
total batches.
Ok, we have the idea of batch size down now, but what's the point? Why not just pass each data element one-by-one to our model rather than grouping the data in batches?
Why use batches?
Well, for one, generally the larger the batch size, the quicker our model will complete each epoch during training. This is because, depending on our computational resources, our machine may be able to process much more than one single sample at a time.
The trade-off, however, is that even if our machine can handle very large batches, the quality of the model may degrade as we set our batch larger and may ultimately cause the model to be unable to generalize well on data it hasn't seen before.
In general, the batch size is another one of the hyperparameters that we must test and tune based on how our specific model is performing during training. This parameter will also have to be tested in regards to how our machine is performing in terms of its resource utilization when using different batch sizes.
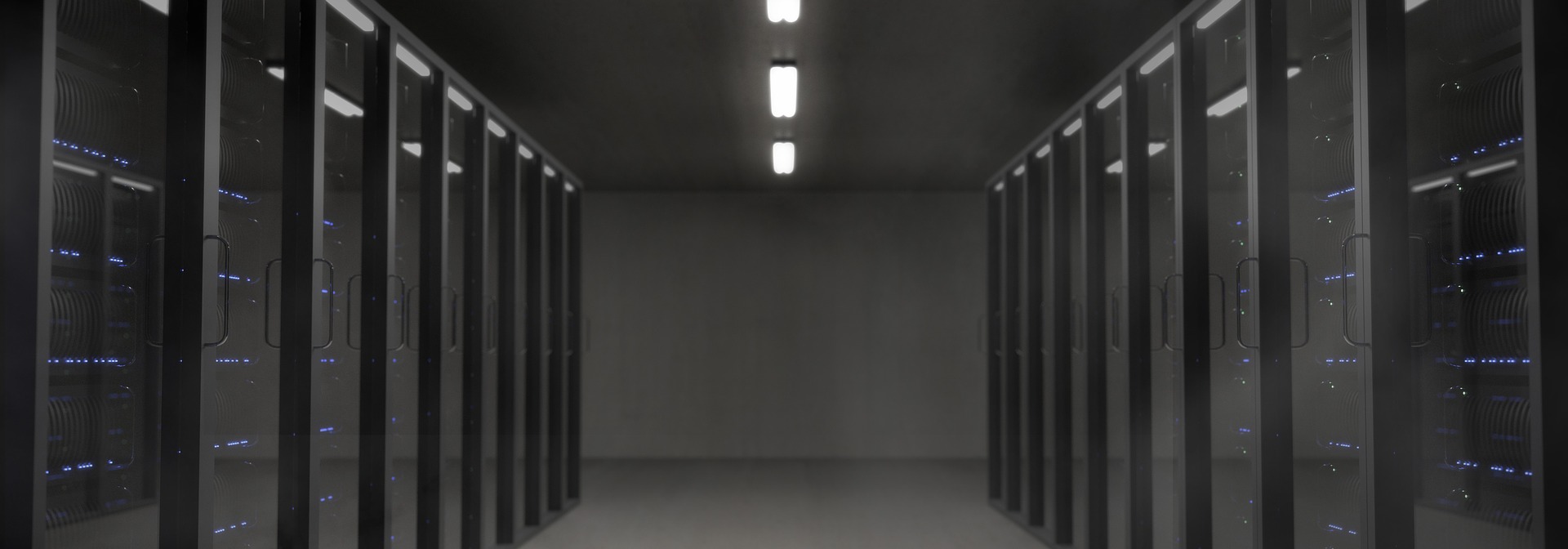
For example, if we were to set our batch size to a relatively high number, say 100
, then our machine may not have enough computational power to process all 100
images in parallel,
and this would suggest that we need to lower our batch size.
Mini-batch gradient descent
Additionally, note if using mini-batch gradient descent, which is normally the type of gradient descent algorithm used by most neural network APIs like Keras by default, the gradient update will occur on a per-batch basis. The size of these batches is determined by the batch size.
This is in contrast to stochastic gradient descent, which implements gradient updates per sample, and batch gradient descent, which implements gradient updates per epoch.
Alright, we should now have a general idea about what batch size is. Let's see how we specify this parameter in code now using Keras.
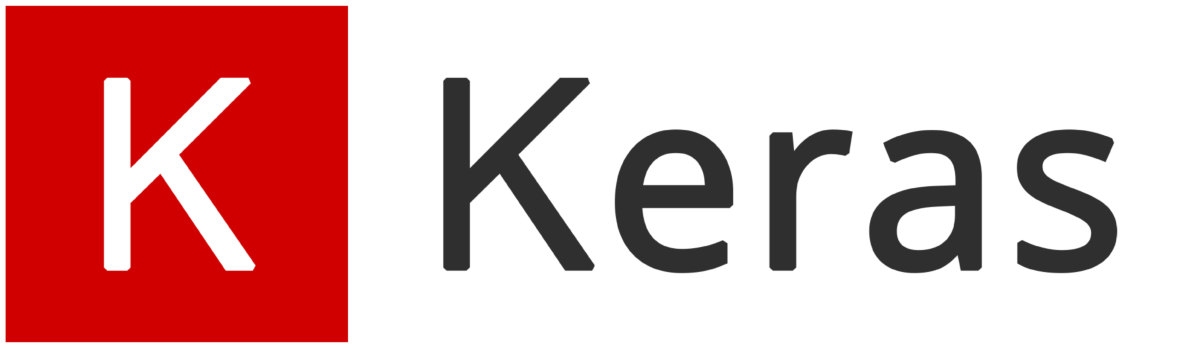
Working with batch size in Keras
We'll be working with the same model we've used in the last several posts. This is just an arbitrary Sequential
model.
model = Sequential([
Dense(units=16, input_shape=(1,), activation='relu'),
Dense(units=32, activation='relu', kernel_regularizer=regularizers.l2(0.01)),
Dense(units=2, activation='sigmoid')
])
Let's focus our attention on where we call model.fit()
. We know this is the function we call to train our model, and we saw this in action in our previous
post
on how an artificial neural network learns.
model.fit(
x=scaled_train_samples,
y=train_labels,
validation_data=valid_set,
batch_size=10,
epochs=20,
shuffle=True,
verbose=2
)
This fit()
function accepts a parameter called batch_size
. This is where we specify our batch_size
for training. In this example, we've just arbitrarily set
the value to 10
.
Now, during the training of this model, we'll be passing in 10
samples at a time until we eventually pass in all the training data to complete one single epoch. Then, we'll start
the same process over again to complete the next epoch.
That's really all there is to it for specifying the batch size for training a model in Keras!
Wrapping up
Hopefully now we have a general understanding of what the batch size is and how to specify it in Keras. I'll see you in the next one!
quiz
resources
updates
Committed by on