Learning Rate in a Neural Network explained
text
Learning rates and neural networks
In this post, we'll be discussing the learning rate, and we'll see how it's used when we train a neural network.
In our previous post on what it means for an artificial neural network to learn, we briefly mentioned the learning rate as a number that we multiply our resulting gradient by. Let's go more into that idea now.
Introducing the learning rate
We know that the objective during training is for SGD to minimize the loss between the actual output and the predicted output from our training samples. The path towards this minimized loss is occurring over several steps.
Recall that we start the training process with arbitrarily set weights, and then we incrementally update these weights as we move closer and closer to the minimized loss.
Now, the size of these steps we're taking to reach our minimized loss is going to depend on the learning rate. Conceptually, we can think of the learning rate of our model as the step size.
Before going further, let's first pause for a quick refresher. We know that during training, after the loss is calculated for our inputs, the gradient of that loss is then calculated with respect to each of the weights in our model.
Once we have the value of these gradients, this is where the idea of our learning rate comes in. The gradients will then get multiplied by the learning rate.
This learning rate is a small number usually ranging between 0.01
and 0.0001
, but the actual value can vary, and any value we get for the gradient is going to become pretty small
once we multiply it by the learning rate.
Updating the network's weights
Alright, so we get the value of this product for each gradient multiplied by the learning rate, and we then take each of these values and update the respective weights by subtracting this value from them.
We ditch the previous weights that were set on each connection and update them with these new values.
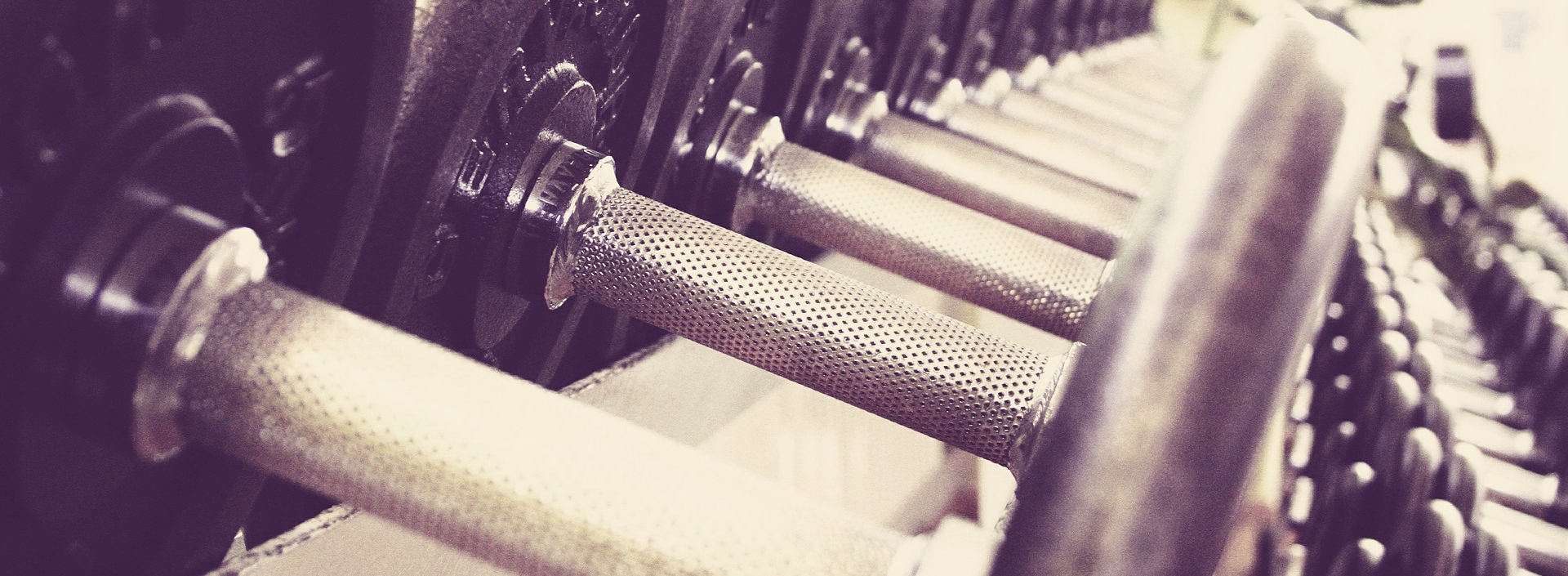
The value we choose for the learning rate is going to require some testing. The learning rate is another one of those
hyperparameters that we have to test and tune with each model before we know exactly where we want to set it, but as mentioned earlier, a typical guideline is to set it somewhere between
0.01
and 0.0001
.
When setting the learning rate to a number on the higher side of this range, we risk the possibility of overshooting. This occurs when we take a step that's too large in the direction of the minimized loss function and shoot past this minimum and miss it.
To avoid this, we can set the learning rate to a number on the lower side of this range. With this option, since our steps will be really small, it will take us a lot longer to reach the point of minimized loss.
Overall, the act of choosing between a higher learning rate and a lower learning rate leaves us with this kind of trade-off idea.
Alright, so now we should have an idea about what the learning rate is and how it fits into the overall process of training.
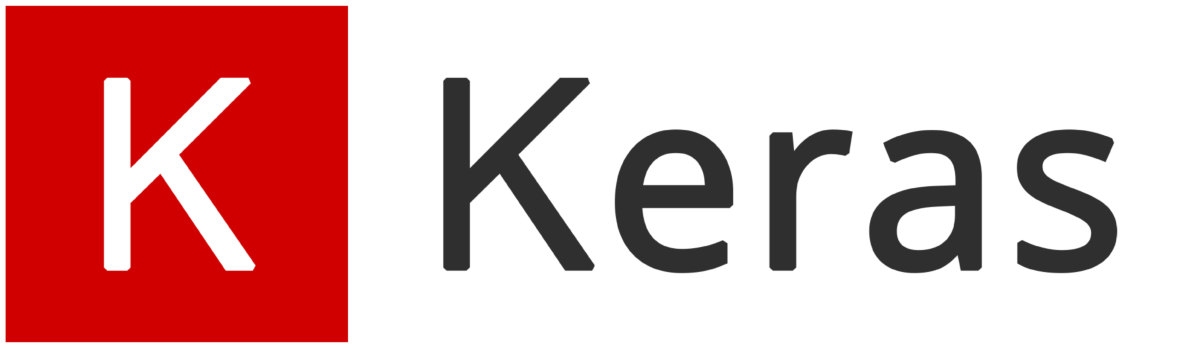
Let's see how we can specify the learning rate in code using Keras.
Learning rates in Keras
This is the model that we've used in previous posts.
model = Sequential([
Dense(units=16, input_shape=(1,), activation='relu'),
Dense(units=32, activation='relu', kernel_regularizer=regularizers.l2(0.01)),
Dense(units=2, activation='sigmoid')
])
model.compile(
optimizer=Adam(learning_rate=0.0001),
loss='sparse_categorical_crossentropy',
metrics=['accuracy']
)
With the line where we're compiling our model, we can see that the first parameter we're specifying is our optimizer. In this case, we're using Adam
as the optimizer for
this model.
Now to our optimizer, we can optionally pass our learning rate by specifying the learning_rate
parameter. We can see that here we're specifying 0.0001
as the learning rate.
We mentioned that this learning_rate
parameter is optional. If we don't explicitly set it, then the default learning rate that Keras has assigned to this particular optimizer will be
set. To see what this default learning rate is, you'll need to check the Keras
documentation for the optimizer you're specifying.
There's also another way we can specify the learning rate. After compiling our model, we can set the learning rate by setting model.optimizer.learning_rate
to our designated value.
model.optimizer.learning_rate = 0.01
Here we can see that we're setting it to 0.01
. Now, if we print the value of our learning rate, we can see it has now changed from .0001
to .01
.
> model.optimizer.learning_rate
0.01
That's all there is to it for specifying the learning rate for our model in Keras.
Wrapping up
We should now have an understanding of what the learning rate is, how it fits into the overall process of training, and why we need to test and tune it to find the value that is just right for our model. I'll see you in the next one!
quiz
resources
updates
Committed by on