Regularization in a Neural Network explained
text
Regularization in a neural network
In this post, we'll discuss what regularization is, and when and why it may be helpful to add it to our model.
In our previous post on overfitting, we briefly introduced dropout and stated that it is a regularization technique. We hadn't yet discussed what regularization is, so let's do that now.
In general, regularization is a technique that helps reduce overfitting or reduce variance in our network by penalizing for complexity. The idea is that certain complexities in our model may make our model unlikely to generalize well, even though the model fits the training data.
Given this, if we add regularization to our model, we're essentially trading in some of the ability of our model to fit the training data well for the ability to have the model generalize better to data it hasn't seen before.
To implement regularization is to simply add a term to our loss function that penalizes for large weights. We'll expand on this idea in just a moment.
L2 regularization
The most common regularization technique is called L2 regularization. We know that regularization basically involves adding a term to our loss function that penalizes for large weights.
L2 regularization term
With L2 regularization, the term we're adding to the loss is the sum of the squared norms of the weight matrices
$$\sum_{j=1}^{n}\left\Vert w^{[j]}\right\Vert ^{2},$$
multiplied by a small constant
$$\frac{\lambda }{2m}.$$
Norms are positive
If you're not familiar with norms in general, understand that a norm is just a function that assigns a strictly positive length or size for each vector in a vector space. The vector space we're working with here depends on the sizes of our weight matrices.
Rather than going on a linear algebra tangent about norms in this moment, we'll continue on with the general idea about regularization. Given that norms are a fundamental concept of linear algebra, there is a lot of information available on the web that explains norms in detail if you need to get a better grasp.
To over simplify, know for now that the norm of each of our weight matrices is just going to be a positive number.
Suppose that \(v\) is a vector in a vector space. The norm of \(v\) is denoted as \(\left\Vert v\right\Vert,\) and it is required that
\[\left\Vert v\right\Vert \geq 0.\]
Adding the term to the loss
Let's look at what L2 regularization looks like. We have
$$loss + \left( \sum_{j=1}^{n}\left\Vert w^{[j]}\right\Vert ^{2}\right)\frac{\lambda }{2m}.$$
The table below gives the definition for each variable in the expression above.
Variable | Definition |
---|---|
\(n\) | Number of layers |
\(w^{[j]}\) | Weight matrix for the \(j^{th}\) layer |
\(m\) | Number of inputs |
\(\lambda\) | Regularization parameter |
The term \(\lambda\) is called the regularization parameter, and this is another hyperparameter that we'll have to choose and then test and tune in order to choose the correct number for our specific model.
To summarize, we now know that regularization is just a technique that penalizes for relatively large weights in our model, and behind the scenes, the implementation of regularization is just the addition of a term to our existing loss function.
Impact of regularization
So why does regularization help?
Well, using L2 regularization as an example, if we were to set \(\lambda\) to be large, then it would incentivize the model to set the weights close to zero because the objective of SGD is to minimize the loss function. Remember our original loss function is now being summed with the sum of the squared matrix norms,
$$\sum_{j=1}^{n}\left\Vert w^{[j]}\right\Vert ^{2},$$
which is multiplied by
$$\frac{\lambda }{2m}.$$
If \(\lambda\) is large, then this term, \(\frac{\lambda }{2m}\), will continue to stay relatively large, and if we're multiplying that by the sum of the squared norms, then the product may be relatively large depending on how large our weights are. This means that our model is incentivized to make the weights small so that the value of this entire function stays relatively small in order to minimize loss.
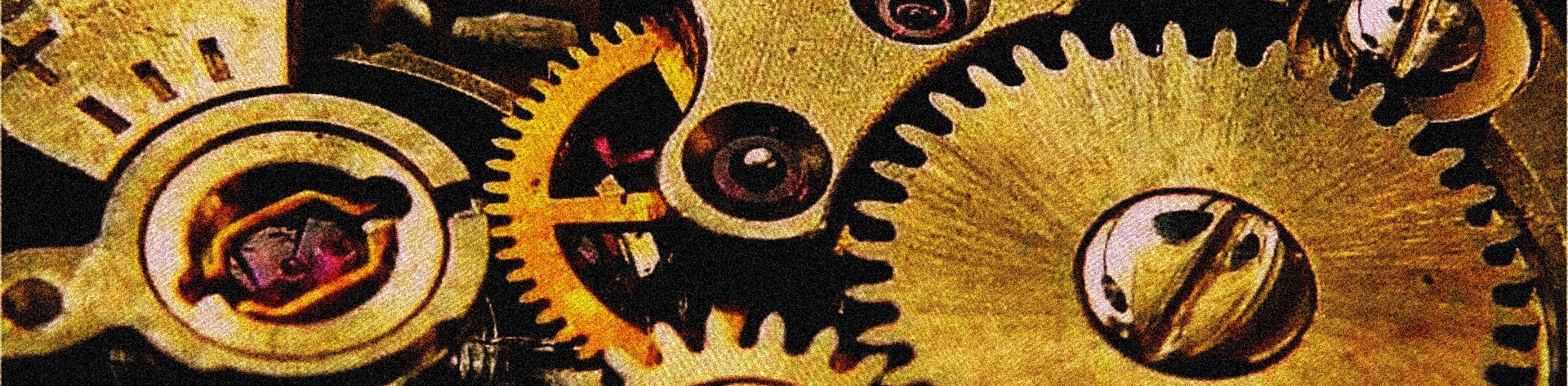
Intuitively, we could think that maybe this technique will set the weights so close to zero, that it could basically zero-out or reduce the impact of some of our layers. If that's the case, then it would conceptually simplify our model, making our model less complex, which may in turn reduce variance and overfitting.
Wrapping up
We should now have a good understanding about what regularization is, its impact, and how L2 regularization works. See ya next time!
quiz
resources
updates
Committed by on