video
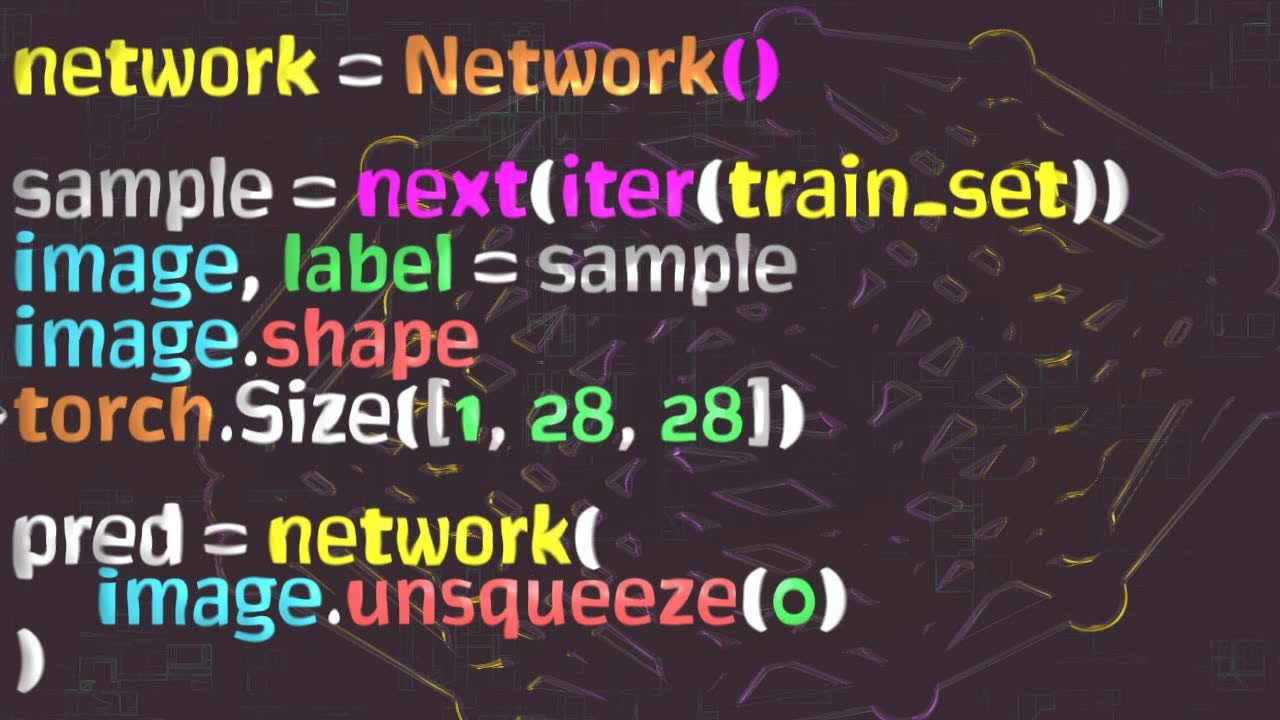
Deep Learning Course - Level: Intermediate
Welcome to this series on neural network programming with PyTorch. In this episode, we will see how we can use our convolutional neural network to generate an output prediction tensor from a sample image of our dataset. Without further ado, let's get started.
At this point in the series, we've finished building our model, and technically, we could jump right into the training process from here. However, let's work to better understand how our network is working right out of the box, and then, once we understand our network a little more deeply, we'll be better prepared to understand the training process.
Committed by on