video
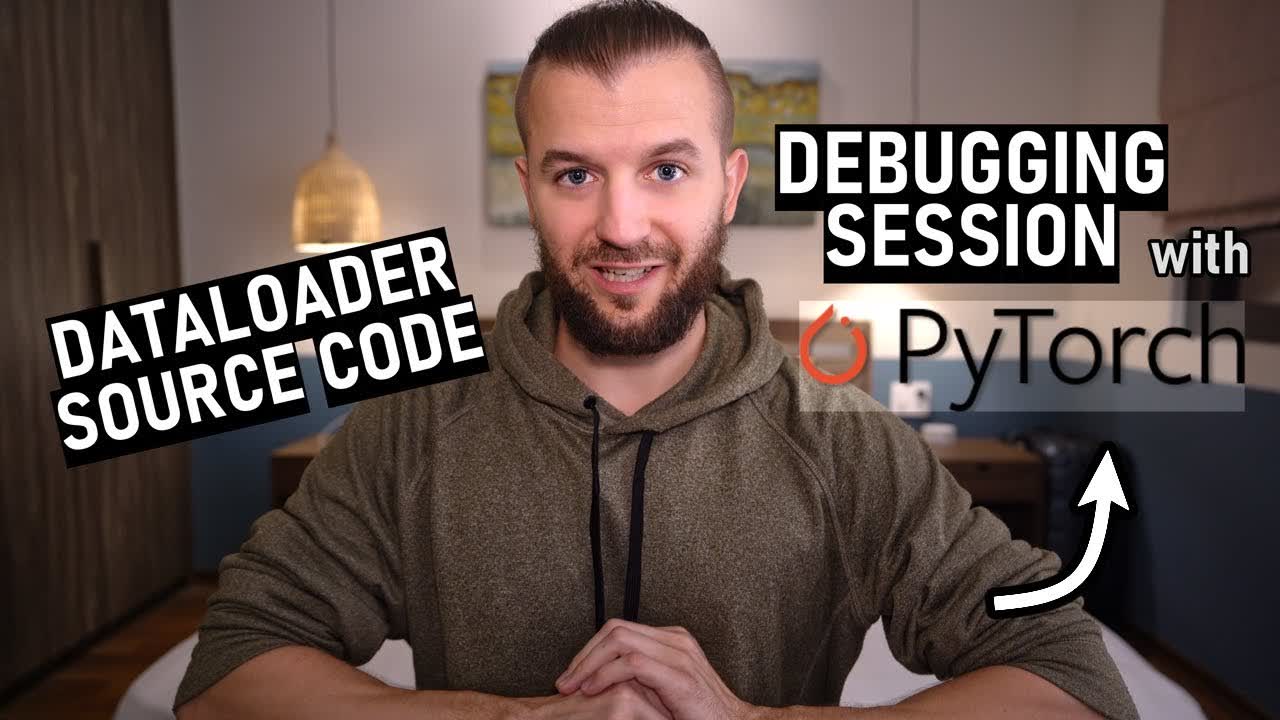
Deep Learning Course - Level: Intermediate
Welcome to DeepLizard. My name's Chris. In this episode, we're going to pick up where we left off last time looking at data normalization. Only this time, instead of writing code, we're going be debugging the code, and specifically, we're going be debugging down into the PyTorch source code to see exactly what's going on when we normalize a data set.
Without further ado, let's get started.
Committed by on