video
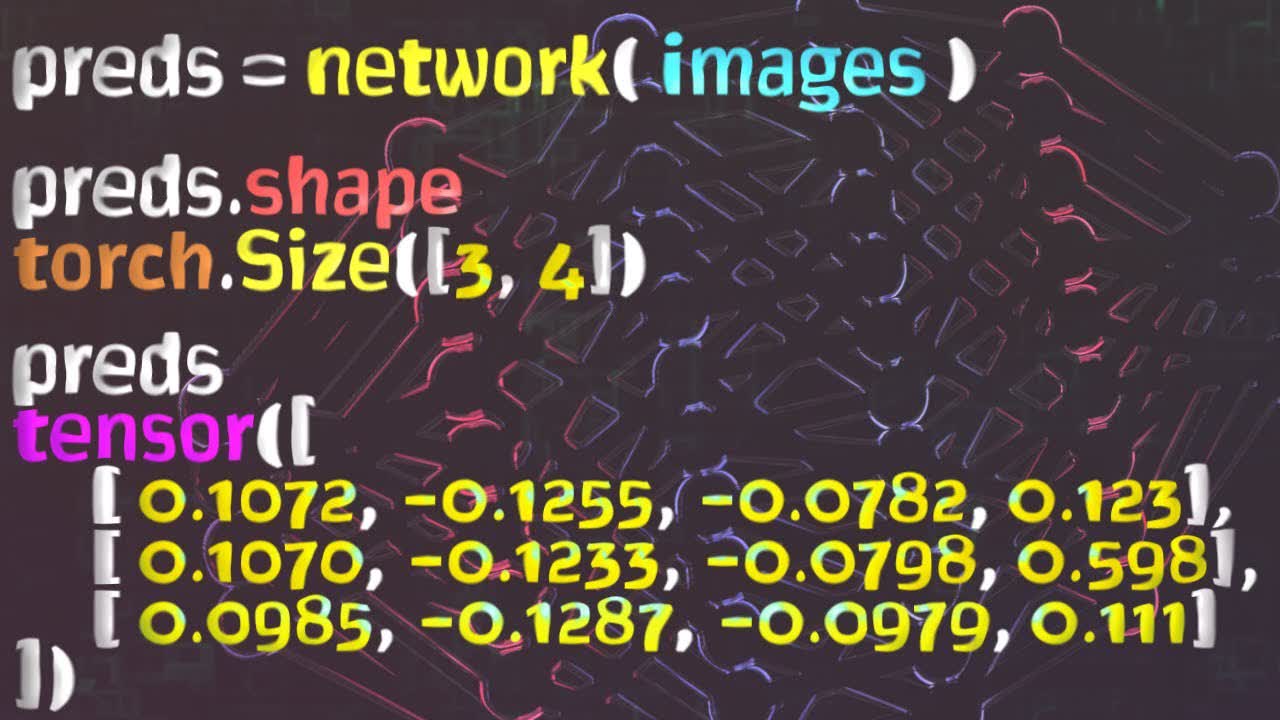
Deep Learning Course - Level: Intermediate
Welcome to this neural network programming series with PyTorch. Our goal in this episode is to pass a batch of images to our network and interpret the results. Without further ado, let's get started.
In the last episode, we learned about forward propagation and how to pass a single image from our training set to our network. Now, let's see how to do this using a batch of images. We'll use the data loader to get the batch, and then, after passing the batch to the network, we'll interpret the output.
Committed by on