video
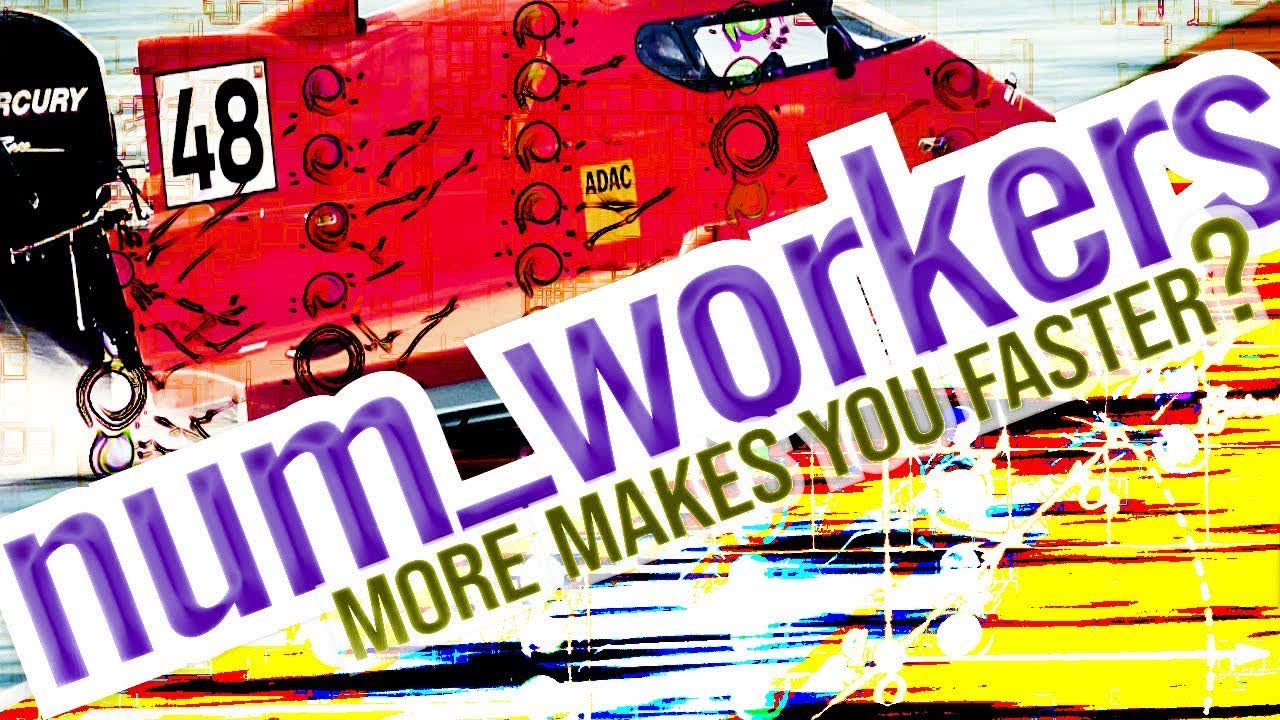
Deep Learning Course - Level: Intermediate
num_workers
Test - Speed Things Up
Welcome to this neural network programming series. In this episode, we will see how we can speed up the neural network training process by utilizing the multiple process capabilities of the PyTorch DataLoader class.
Without further ado, let's get started.
Committed by on