video
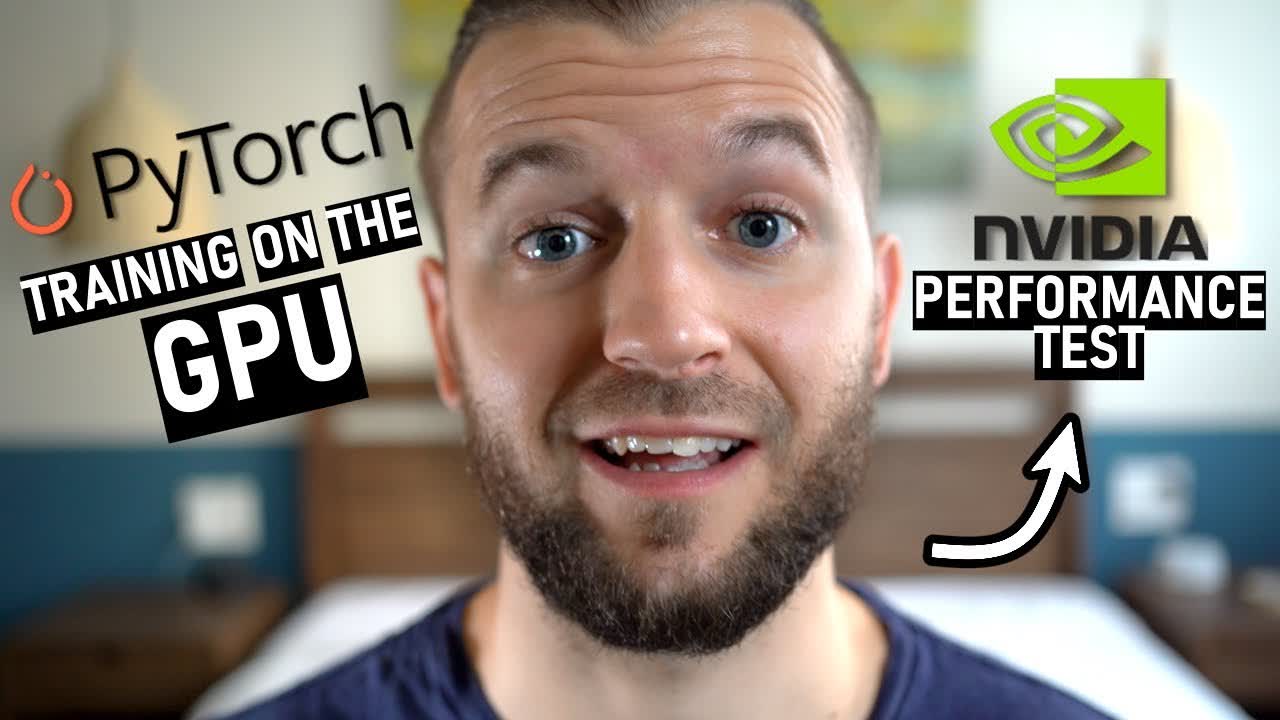
Deep Learning Course - Level: Intermediate
Welcome to deeplizard. My name is Chris. In this episode, we're going to learn how to use the GPU with PyTorch. We'll see how to use the GPU in general, and we'll see how to apply these general techniques to training our neural network.
Without further ado, let's get started.
Committed by on