video
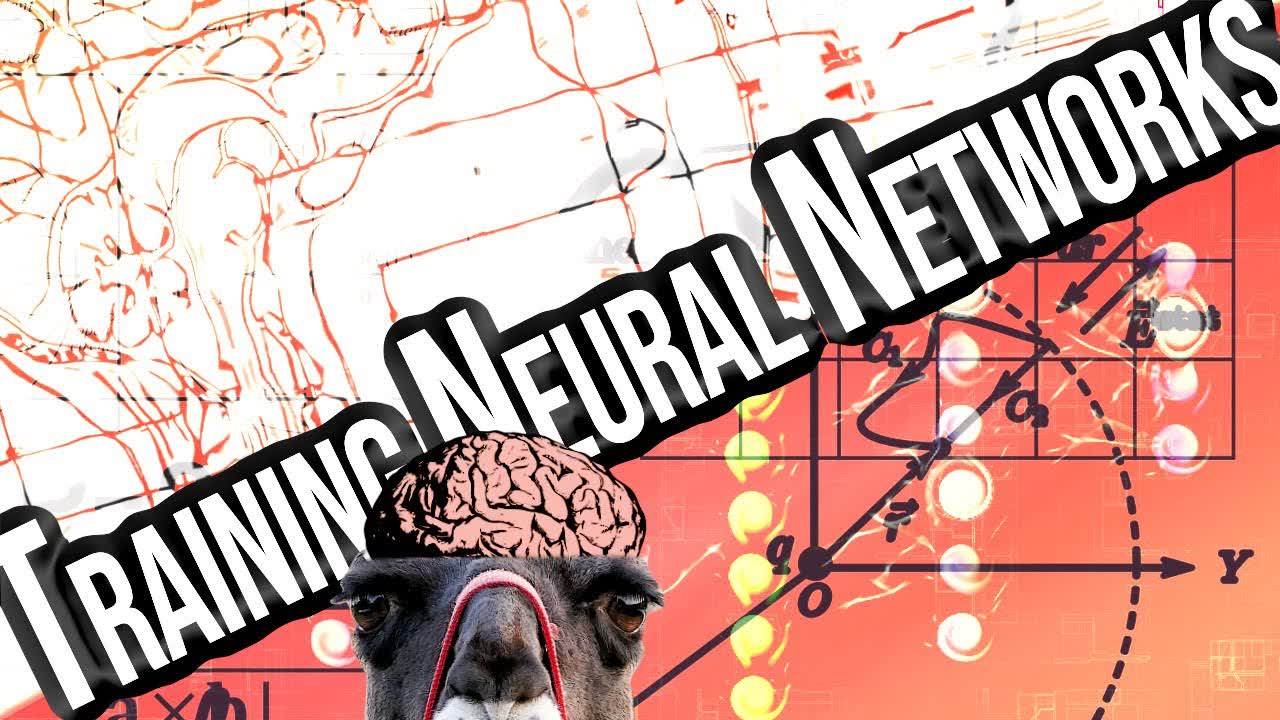
Deep Learning Course - Level: Intermediate
Welcome to this neural network programming series with PyTorch. In this episode, we will learn the steps needed to train a convolutional neural network.
So far in this series, we learned about Tensors, and we've learned all about PyTorch neural networks. We are now ready to begin the training process.
Committed by on