video
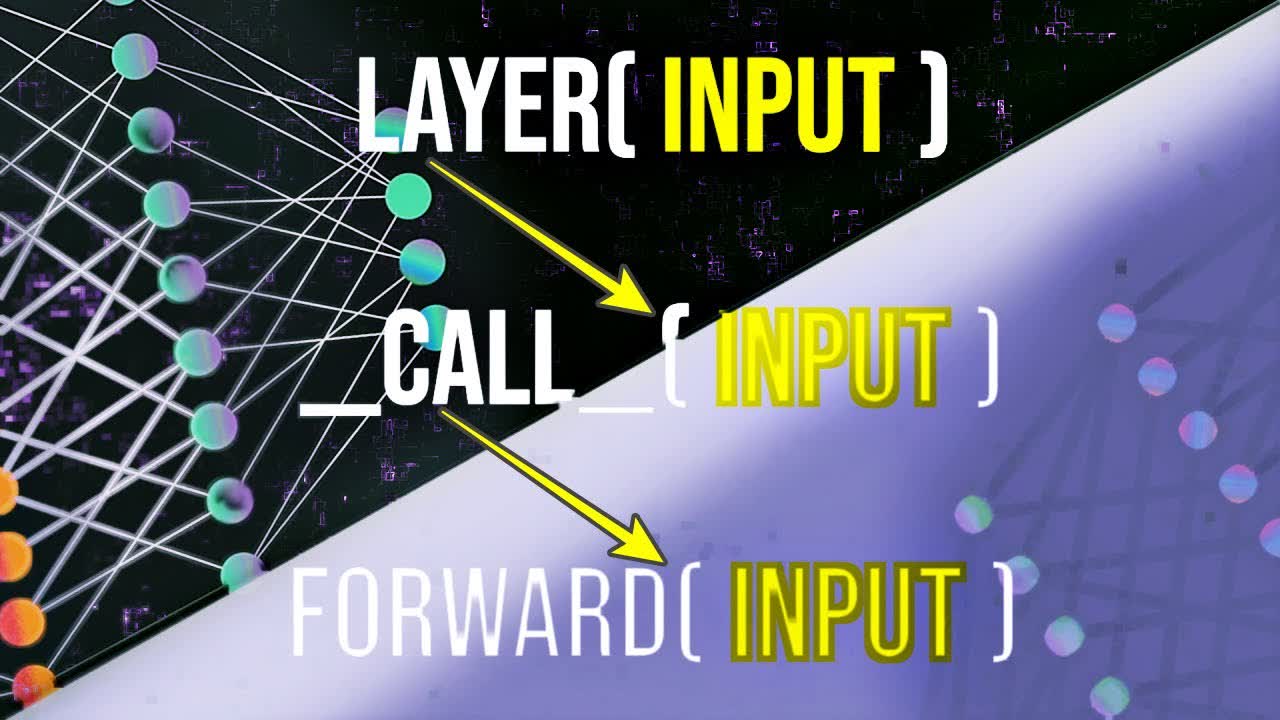
Deep Learning Course - Level: Intermediate
Welcome to this series on neural network programming with PyTorch. In this one, we'll learn about how PyTorch neural network modules are callable, what this means, and how it informs us about how our network and layer forward methods are called.
Without further ado, let's get started.
Committed by on